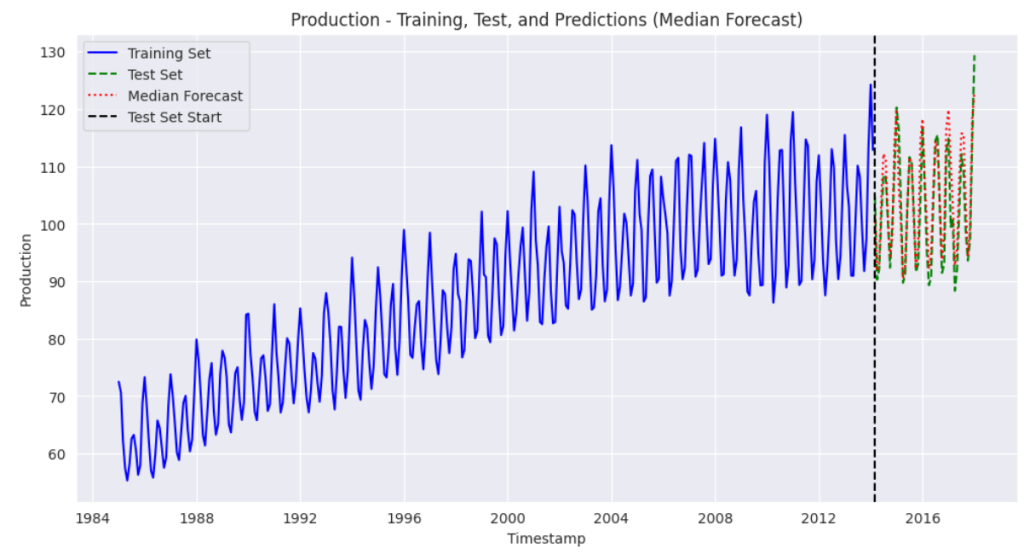
Blog
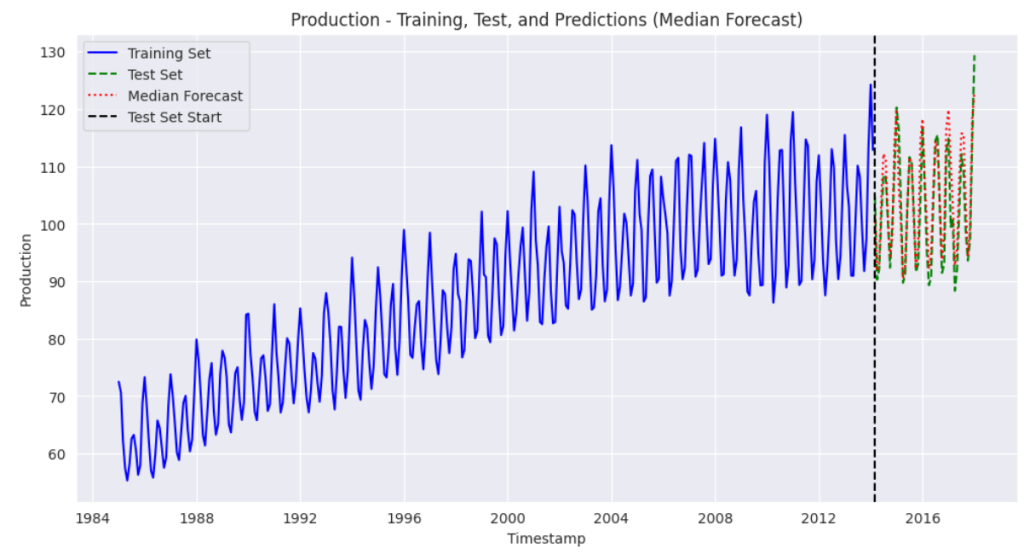
Time Series Classification with Amazon Chronos Model with GridDB
This article shows how to build a time series forecasting model for electricity production using Amazon Chronos and GridDB database. We will retrieve historical electricity
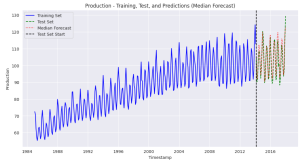
This article shows how to build a time series forecasting model for electricity production using Amazon Chronos and GridDB database. We will retrieve historical electricity production data from Kaggle, insert it into a GridDB time series container, and use the data to train a forecasting model with Amazon Chronos, a specialized collection of time series models based on the T5 architecture. GridDB is a robust NOSQL database optimized for efficiently handling large volumes of real-time data. Its advanced in-memory processing and time series data management make it ideal for big data and IoT applications. GridDB’s real-time data processing capabilities and Chronos’ state-of-the-art forecasting methods make them a powerful combination for time forecasting applications. Prerequisites You need to install the following libraries to run codes in this article. GridDB C Client GridDB Python client Instructions for installing these clients are available on GridDB Python Package Index (Pypi). You must also install Amazon Chronos, Numpy, Pandas, and Matplotlib libraries. The scripts below will help you install and import the necessary libraries for running codes. %pip install git+https://github.com/amazon-science/chronos-forecasting.git %pip install matplotlib seaborn numpy pandas scikit-learn import matplotlib.pyplot as plt from matplotlib.dates import DateFormatter import seaborn as sns import numpy as np import pandas as pd import torch from chronos import ChronosPipeline import griddb_python as griddb from sklearn.metrics import mean_absolute_error Inserting Time Series Data Into GriddB The first step is to insert the time series data we want to forecast into GridDB. This section explains the steps involved. Downloading and Importing Electricity Production Data from Kaggle We will forecast future electricity production requirements using the Electricity Production dataset from Kaggle. The following script imports the CSV file containing the dataset into a pandas DataFrame. dataset = pd.read_csv(“Electric_Production.csv”) dataset.head(10) Output: The dataset consists of monthly electricity production from 1st January 1985 to 1st January 2018. You can draw line plots to see that electricity production highly depends on the month of the year. # Create the line plot sns.set_style(“darkgrid”) plt.figure(figsize=(12, 7)) sns.lineplot(data=dataset, x=’DATE’, y=’IPG2211A2N’, label=’Electricity Production’) plt.xlabel(‘Date’) plt.ylabel(‘Electricity Production (IPG2211A2N)’) plt.title(‘Electricity Production Over Time’) plt.legend() plt.show() Output: Once we have our dataset, we can insert this data into GridDB. Connect to GriddB To connect to GridDB, you need to create an object of the StoreFactory class. Next, call the get_store() method on the store factory object and pass the DB host and cluster names, user, and password. To test if the connection is successful, call the get_container() method and pass it the name of any container. If you see the following output, your connection is successful. # GridDB connection details DB_HOST = “127.0.0.1:10001” DB_CLUSTER = “myCluster” DB_USER = “admin” DB_PASS = “admin” # creating a connection factory = griddb.StoreFactory.get_instance() try: gridstore = factory.get_store( notification_member = DB_HOST, cluster_name = DB_CLUSTER, username = DB_USER, password = DB_PASS ) container1 = gridstore.get_container(“container1”) if container1 == None: print(“Container does not exist”) print(“Successfully connected to GridDB”) except griddb.GSException as e: for i in range(e.get_error_stack_size()): print(“[“, i, “]”) print(e.get_error_code(i)) print(e.get_location(i)) print(e.get_message(i)) Output: Container does not exist Successfully connected to GridDB Create Container for Electricity Production Data in GridDB GrirDB stores data containers. You need the container name and column information to create a container. You can assign any name to your container. However, the container information must be a list of lists, each nested list containing the column name and the column type. For example, in the script below we have two columns: TimeStamp with column type griddb.Type.TIMESTAP, and Production with griddb.Type.DOUBLE type. Next, you need to create an object of the ContainerInfo class and pass the container name and column info to the ContainerInfo class constructor. Finally, call the put_container() method and pass to it the ContainerInfo class object to create a container in the GridDB. dataset[‘DATE’] = pd.to_datetime(dataset[‘DATE’]) container_name = “Electricity_Production” column_info = [ [“Timestamp”, griddb.Type.TIMESTAMP], [“Production”, griddb.Type.DOUBLE] ] container_info = griddb.ContainerInfo(container_name, column_info, griddb.ContainerType.TIME_SERIES) # Creating Container try: gridstore.put_container(container_info) container = gridstore.get_container(container_name) if container is None: print(f”Failed to create container: {container_name}”) else: print(f”Successfully created container: {container_name}”) except griddb.GSException as e: print(f”Error creating or retrieving container {container_name}:”) for i in range(e.get_error_stack_size()): print(f”[{i}]”) print(f”Error code: {e.get_error_code(i)}”) print(f”Location: {e.get_location(i)}”) print(f”Message: {e.get_message(i)}”) Output Successfully created container: Electricity_Production You can retrieve the container you created using the get_container() method. The next step is to store our dataset in the container we just created. Insert Electricity Production Data into GridDB Container To insert data into our GridDB container, we will iterate through all the rows in our dataset, call our container object’s put() method, and pass the values from the DATE and IPG2211A2N columns to the method. try: for index, row in dataset.iterrows(): container.put([row[‘DATE’].to_pydatetime(), row[‘IPG2211A2N’]]) print(f”Successfully inserted {len(dataset)} rows of data into {container_name}”) except griddb.GSException as e: print(f”Error inserting data into container {container_name}:”) for i in range(e.get_error_stack_size()): print(f”[{i}]”) print(f”Error code: {e.get_error_code(i)}”) print(f”Location: {e.get_location(i)}”) print(f”Message: {e.get_message(i)}”) Output Successfully inserted 397 rows of data into Electricity_Production We have successfully inserted the electricity production data into the GridDB. The next step is to forecast electricity production using Amazon’s Chronos model. Forecasting Electricity Production using Amazon’s Chronos Time Series Model Amazon Chronos is a collection of pre-trained language models specifically designed for time series forecasting. These models are based on the T5 (Text-to-Text Transfer Transformer) architecture, which has been adapted to handle time series data. Retrieving Data from GridDB We first need to retrieve data we stored in GridDB to forecast electricity production. To do so, you can use the get_container() method and pass it the container name you want to retrieve. Call the SELECT * query using the container’s query() method. Next, call the fetch() method to retrieve the dataset object. Finally, call the fetch_rows() method to store the dataset into a pandas DataFrame. def retrieve_data_from_griddb(container_name): try: stock_data_container = gridstore.get_container(container_name) # Query all data from the container query = stock_data_container.query(“select *”) rs = query.fetch() # Adjust the number based on your data size data = rs.fetch_rows() data .set_index(“Timestamp”, inplace=True) return data except griddb.GSException as e: print(f”Error retrieving data from GridDB: {e.get_message()}”) return None electric_production_data = retrieve_data_from_griddb(“Electricity_Production”) electric_production_data.head() Output: Forecasting Electricity Production using Amazon Chronos Model Amazon Chronos models are available for free on Hugging Face. For making inferences, you can simply install the model from GitHub. We will divide our dataset into a training and test set. Then, we will use the Amazon Chronos model to forecast electricity production for the months in the test set. Finally, we will evaluate the model’s performance by comparing the forecasted electricity production with the actual production. The following script divides the dataset into train and test sets. The dataset has a total of 397 records. We will use the last 47 records for testing. # Define the test size and calculate the split index test_size = 47 split_index = len(electric_production_data) – test_size # Check if the data length is shorter than the test size if split_index < 0: train_production = pd.Series(dtype=float) test_production = electric_production_data[‘Production’] else: # Splitting the Production column into training and test sets train_production = electric_production_data[‘Production’].iloc[:split_index] test_production = electric_production_data[‘Production’].iloc[split_index:] # Display the results print(“Training Set:”) print(train_production.shape) print(“\nTest Set:”) print(test_production.shape) Output: Training Set: (350,) Test Set: (47,) Next, we will import the pretrained Chronos t5 large model using the ChronosPipeline.from_pretrained() method. pipeline = ChronosPipeline.from_pretrained( “amazon/chronos-t5-large”, device_map=”cuda”, torch_dtype=torch.bfloat16, ) Chronos models expect data to be in torch tensor format. The script below converts the data into torch tensors. Next, we use the pipeline.predict() method to forecast the next 47 months of electricity production based on the training data (context). We divide the predictions into three quantiles (0.1, 0.5, 0.9). context = torch.tensor(train_production) prediction_length = test_size forecast = pipeline.predict(context, prediction_length) low, median, high = np.quantile(forecast[0].numpy(), [0.1, 0.5, 0.9], axis=0) Next, we evaluate the model performance. Evaluating Model Performance We will plot the median forecast values against the test values. To do so, we will create a pandas DataFrame that contains our predictions. The following script plots the training set, test set, and predictions. test_production.index = pd.to_datetime(test_production.index) median_forecast = pd.Series(median, index=test_production.index, name=”Median Forecast”) plt.figure(figsize=(12, 6)) plt.plot(train_production.index, train_production, color=’blue’, label=”Training Set”, linestyle=”-“) plt.plot(test_production.index, test_production, color=’green’, linestyle=”–“, label=”Test Set”) plt.plot(median_forecast.index, median_forecast, color=’red’, linestyle=”:”, label=”Median Forecast”) # Vertical line to mark the start of the test set plt.axvline(x=test_production.index[0], color=’black’, linestyle=”–“, label=”Test Set Start”) plt.xlabel(“Timestamp”) plt.ylabel(“Production”) plt.title(“Production – Training, Test, and Predictions (Median Forecast)”) plt.legend() plt.show() Output: The above output shows that our model performs well and can capture the trends in the training dataset. The predictions are close to the values in the test set. Next, we will plot only the test values against the median prediction values and the 80% prediction interval. median_forecast = pd.Series(median, index=test_production.index, name=”Median Forecast”) lower_bound = pd.Series(low, index=test_production.index, name=”Lower Bound”) upper_bound = pd.Series(high, index=test_production.index, name=”Upper Bound”) plt.figure(figsize=(12, 6)) plt.plot(test_production.index, test_production, color=’green’, linestyle=”:”, label=”Actual Production”) plt.plot(median_forecast.index, median_forecast, color=’red’, linestyle=”:”, label=”Median Forecast”) # Plot the 80% prediction interval as an orange shaded area plt.fill_between(test_production.index, lower_bound, upper_bound, color=’orange’, alpha=0.3, label=”80% Prediction Interval”) plt.xlabel(“Timestamp”) plt.ylabel(“Production”) plt.title(“Production – Actual vs. Forecast with 80% Prediction Interval”) plt.legend() plt.show() Output: The above output shows that prediction values in 80% intervals cover almost all the test data, showing that our model performs exceptionally well for forecasting time series data. Finally, we will plot the mean absolute error (MAE) values for the predictions to quantify the results. mae = mean_absolute_error(test_production, median_forecast) # Print results print(“Average electricity production values in the training set:”, train_production.mean()) print(“Mean Absolute Error (MAE):”, mae) Output: Average electricity production values in the training set: 86.9775362857143 Mean Absolute Error (MAE): 3.2303302385930803 We obtain an MAE value of 3.23, indicating that, on average, our model’s predictions are only 3.23 units off from the actual test values, just a 3.4% deviation from the average electricity production in the training set. Conclusion This article covered the complete workflow for time series forecasting with GridDB and Amazon Chronos. You saw how to connect to GridDB, insert time series data, and train an Amazon Chronos model to forecast electricity production. The results showed accurate predictions, capturing seasonal trends and providing reliable forecasts within an 80% confidence interval. Combining GridDB’s robust time series data management with Chronos’ specialized forecasting models provides a scalable solution for accurate time-series
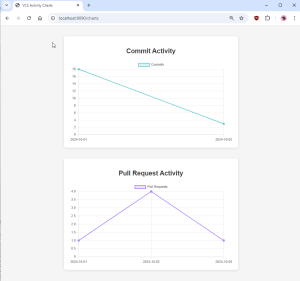
Introduction In today’s competitive landscape, efficient software development is crucial for delivering high-quality products. A key factor in achieving this is monitoring developer activity in Version Control Systems (VCS) to optimize collaboration and code quality. By analyzing patterns in code commits, pull requests, merges, and branching, organizations can identify bottlenecks, improve workflows, and ultimately boost productivity. Popular VCS tools such as Git, SVN, and GitLab allow teams to track changes, but analyzing large-scale development activities over time can be challenging. This is where GridDB, a high-performance time-series database, proves invaluable. Specifically designed to manage vast amounts of time-sensitive data, GridDB enables teams to efficiently capture, store, and analyze VCS events. Why Use GridDB for VCS Activity Monitoring? Time-Stamped Data Handling Each commit, pull request, and merge event in a VCS is tied to a specific timestamp. GridDB is optimized for time-series data, making it ideal for capturing and analyzing time-sensitive developer activities. Scalability In large, distributed teams, thousands of commits and pull requests may occur daily. GridDB’s scalable architecture ensures that even large volumes of VCS events can be ingested and queried efficiently, without performance degradation. Efficient Data Retrieval GridDB allows time-based queries to quickly retrieve developer activities over specific periods (e.g., daily, weekly, monthly), providing real-time insights into team productivity and collaboration. Historical Insights By analyzing past events—such as spikes in code conflicts or periods of low activity—teams can uncover patterns that lead to more efficient workflows. GridDB’s ability to store and query historical data facilitates in-depth analysis of development trends. In this blog, we’ll explore how to monitor Version Control System (VCS) Activity using GridDB with a simple spring boot project. Specifically, we’ll track key development events such as: Code commits Pull requests Code merges By storing and analyzing this data with GridDB, software teams, and project managers can: Monitor developer productivity by tracking code changes over time. Identify patterns such as merge conflicts or periods of high/low activity. Optimize collaboration by evaluating branching strategies and pull request workflows. Perform historical analysis to improve long-term development trends and practices. Setting Up GridDB Cluster and Spring Boot Integration: For Real-Time Monitoring The first step is to set up a GridDB cluster and integrate it with our Spring Boot application as follows. Source Code for this Project You can clone the GridDBnet blogs repo and grab the apporipriate branch for the source code. $ git clone https://github.com/griddbnet/Blogs.git –branch vcs Setting up GridDB Cluster GridDB provides flexible options to meet different requirements. For development, a single-node cluster on our local machine may be sufficient. However, in production, distributed clusters across multiple machines are typically preferred for improved fault tolerance and scalability. For detailed guidance on setting up clusters based on our deployment strategy, refer to the GridDB documentation. To set up a GridDB cluster, follow the steps mentioned here. Setting up Spring Boot Application Once ourGridDB cluster is operational, the next step is connecting it to ourSpring Boot application. The GridDB Java Client API provides the necessary tools to establish this connection. To simplify the process, you can include the griddb-spring-boot-starter library as a dependency in our project, which offers pre-configured beans for a streamlined connection setup. Project Structure Here’s a suggested project structure for such an application: ├───my-griddb-app │ │ pom.xml │ │ │ ├───src │ │ ├───main │ │ │ ├───java │ │ │ │ └───mycode │ │ │ │ │ MySpringBootApplication.java │ │ │ │ │ │ │ │ │ ├───config │ │ │ │ │ GridDBConfig.java │ │ │ │ │ │ │ │ │ ├───controller │ │ │ │ │ ChartController.java │ │ │ │ │ │ │ │ │ ├───dto │ │ │ │ │ VcsActivityDTO.java │ │ │ │ │ │ │ │ │ └───service │ │ │ │ ChartService.java │ │ │ │ MetricsCollectionService.java │ │ │ │ RestTemplateConfig.java │ │ │ │ │ │ │ └───resources │ │ │ │ application.properties │ │ │ │ │ │ │ └───templates │ │ │ charts.html This structure separates controllers, models, repositories, services, and the application entry point into distinct layers, enhancing modularity and maintainability. Add GridDB Dependency To enable interaction with GridDB in our Spring Boot project, we must include the GridDB Java Client API dependency. This can be accomplished by adding the appropriate configuration to the project build file, such as pom.xml for Maven or the equivalent file for Gradle. Here’s an example of how to configure the dependency in thepom.xml file: <project xmlns=”http://maven.apache.org/POM/4.0.0″ xmlns:xsi=”http://www.w3.org/2001/XMLSchema-instance” xsi:schemaLocation=”http://maven.apache.org/POM/4.0.0 http://maven.apache.org/maven-v4_0_0.xsd”> <modelVersion>4.0.0</modelVersion> <groupId>com.example</groupId> <artifactId>my-griddb-app</artifactId> <version>1.0-SNAPSHOT</version> <name>my-griddb-app</name> <url>http://maven.apache.org</url> <parent> <groupId>org.springframework.boot</groupId> <artifactId>spring-boot-starter-parent</artifactId> <version>3.2.4</version> <relativePath /> <!– lookup parent from repository –> </parent> <properties> <maven.compiler.source>17</maven.compiler.source> <maven.compiler.target>17</maven.compiler.target> </properties> <dependencies> <dependency> <groupId>org.springframework.boot</groupId> <artifactId>spring-boot-starter-actuator</artifactId> </dependency> <dependency> <groupId>junit</groupId> <artifactId>junit</artifactId> <version>3.8.1</version> <scope>test</scope> </dependency> <dependency> <groupId>com.github.griddb</groupId> <artifactId>gridstore</artifactId> <version>5.6.0</version> </dependency> <!– Spring Boot dependencies –> <dependency> <groupId>org.springframework.boot</groupId> <artifactId>spring-boot-starter-web</artifactId> <exclusions> <exclusion> <groupId>org.springframework.boot</groupId> <artifactId>spring-boot-starter-logging</artifactId> </exclusion> </exclusions> </dependency> <dependency> <groupId>org.springframework.boot</groupId> <artifactId>spring-boot-starter-test</artifactId> <scope>test</scope> </dependency> <dependency> <groupId>org.springframework.boot</groupId> <artifactId>spring-boot-starter-thymeleaf</artifactId> </dependency> <!– JSON processing –> <dependency> <groupId>com.fasterxml.jackson.core</groupId> <artifactId>jackson-databind</artifactId> <version>2.15.0</version> <!– or the latest version –> </dependency> <!– Lombok –> <dependency> <groupId>org.projectlombok</groupId> <artifactId>lombok</artifactId> <optional>true</optional> </dependency> </dependencies> </project> Generate GitHub API Access Token To access GitHub data such as commits and pull requests, you’ll need a GitHub Personal Access Token. Follow the instructions in the official GitHub documentation to create one: Log in to GitHub. Navigate to the GitHub Personal Access Token Documentation. Follow the steps to generate a token with the necessary scopes (repo, user). Configure GridDB Connection After adding the GridDB dependency, the next step is configuring the connection details for our GridDB cluster in our Spring Boot application. This is usually configured in the application.properties file, where you can specify various settings for the application. Here’s a quick example of how to set up those connection details: GRIDDB_NOTIFICATION_MEMBER=127.0.0.1:10001 GRIDDB_CLUSTER_NAME=myCluster GRIDDB_USER=admin GRIDDB_PASSWORD=admin management.endpoints.web.exposure.include=* server.port=9090 # GitHub API token and configuration github.api.token=github_DXXXXXXXXXXXXA5OyZwdXr github.api.base-url=https://api.github.com griddb.cluster.host: The hostname or IP address of ourGridDB cluster. griddb.cluster.port: The port number on which the GridDB cluster is listening. griddb.cluster.user: The username for accessing the GridDB cluster. griddb.cluster.password: The password for the specified GridDB user (replace with ouractual password). server.port=9090: Sets the port on which ourSpring Boot application will run. github.api.token: The personal access token for authenticating with the GitHub API, as generated github.api.base-url: The base URL for making requests to the GitHub API (e.g., https://api.github.com). Create GridDB Client Bean To interact effectively with GridDB in our Spring Boot application,we need to create a dedicated Spring Bean to manage the GridDB connection. This bean will establish the connection using the parameters defined in the application.properties file and will act as the central interface for interacting with the GridDB cluster across the application. Here’s an example of how to define this bean in a Java class named GridDbConfig.java: package mycode.config; import java.util.Properties; import org.springframework.beans.factory.annotation.Value; import org.springframework.context.annotation.Bean; import org.springframework.context.annotation.Configuration; import org.springframework.context.annotation.PropertySource; import com.toshiba.mwcloud.gs.GSException; import com.toshiba.mwcloud.gs.GridStore; import com.toshiba.mwcloud.gs.GridStoreFactory; @Configuration @PropertySource(“classpath:application.properties”) public class GridDBConfig { @Value(“${GRIDDB_NOTIFICATION_MEMBER}”) private String notificationMember; @Value(“${GRIDDB_CLUSTER_NAME}”) private String clusterName; @Value(“${GRIDDB_USER}”) private String user; @Value(“${GRIDDB_PASSWORD}”) private String password; @Bean public GridStore gridStore() throws GSException { // Acquiring a GridStore instance Properties properties = new Properties(); properties.setProperty(“notificationMember”, notificationMember); properties.setProperty(“clusterName”, clusterName); properties.setProperty(“user”, user); properties.setProperty(“password”, password); return GridStoreFactory.getInstance().getGridStore(properties); } } Metric Collection Next, we will focus on collecting, transforming, and storing GitHub data for real-time VCS activity monitoring. The key metrics we’ll be tracking include commits, pull requests, and other GitHub events that provide insights into developer productivity and project activity. To collect the necessary data, we utilize specific API endpoints provided by GitHub. These endpoints allow us to track various development activities: Commits: Accessed via the endpoint GET /repos/{owner}/{repo}/commits, this provides a list of commits in a specified repository. Pull Requests: The endpoint GET /repos/{owner}/{repo}/pulls returns information on pull requests, including their current status. Repository Events: We can retrieve a comprehensive list of events using the endpoint GET /repos/{owner}/{repo}/events. This encompasses all significant actions within the repository. Loading Data into GridDB Once the required data is collected from GitHub, the next step is to load this information into GridDB for storage and further analysis. Here’s a high-level overview of the process: Here’s a high-level overview of the process: Authentication: We begin by authenticating with GitHub’s API using an access token. This token ensures that our requests are authorized and can retrieve the necessary data. Therefore, we will set the header as follows for our API calls. private HttpHeaders createHeaders() { HttpHeaders headers = new HttpHeaders(); headers.set(“Authorization”, “Bearer ” + githubApiToken); return headers; } Data Transformation and Mapping: After retrieval, the data will undergo transformation to align with the schema defined in GridDB. This step involves cleaning the data, filtering out irrelevant information, and mapping it to the appropriate fields within GridDB. Here is our VcsActivityDTOwhich is how our data will be stored in db. package mycode.dto; import lombok.AllArgsConstructor; import lombok.Data; import lombok.NoArgsConstructor; import java.util.Date; import com.toshiba.mwcloud.gs.RowKey; @Data @NoArgsConstructor @AllArgsConstructor public class VcsActivityDTO { @RowKey public Date timestamp; // Time of the activity private String eventType; // Event type: commit, pull request, merge, branch private String developerId; // Developer who performed the activity private String repositoryId; // Repository ID or name private String branch; // Branch associated with the activity private String status; // Status (e.g., success, open, merged, conflict) } Data Ingestion: Finally, the transformed data will be loaded into GridDB, where it can be accessed for real-time monitoring and analysis of developer activities. We will insert the data into our database as follows: TimeSeries ts = store.putTimeSeries(“vcsData”, VcsActivityDTO.class); for (VcsActivityDTO activity : result) { ts.append(activity); } Below is the complete MetricsCollectionService.java, which implements all the aforementioned steps. package mycode.service; import java.util.ArrayList; import org.springframework.beans.factory.annotation.Autowired; import org.springframework.beans.factory.annotation.Value; import org.springframework.http.HttpEntity; import org.springframework.http.HttpHeaders; import org.springframework.http.HttpMethod; import org.springframework.http.ResponseEntity; import org.springframework.scheduling.annotation.Scheduled; import org.springframework.stereotype.Service; import org.springframework.web.client.RestTemplate; import org.springframework.web.util.UriComponentsBuilder; import com.fasterxml.jackson.core.JsonProcessingException; import com.fasterxml.jackson.databind.JsonMappingException; import com.toshiba.mwcloud.gs.*; import mycode.dto.VcsActivityDTO; import java.text.ParseException; import java.text.SimpleDateFormat; import java.util.List; import java.util.Map; @Service public class MetricsCollectionService { @Autowired GridStore store; @Autowired RestTemplate restTemplate; @Value(“${github.api.token}”) private String githubApiToken; @Value(“${github.api.base-url}”) private String githubBaseUrl; @Scheduled(fixedRate = 2222260) // Collect metrics every minute public void collectMetrics() throws GSException, JsonMappingException, JsonProcessingException, ParseException { String repoOwner = “microsoft”; String repoName = “vscode”; List commits = getCommits(repoOwner, repoName); List pullRequests = getPullRequests(repoOwner, repoName); List branchEvents = getBranchEvents(repoOwner, repoName); System.out.println(“Collected Commits: ” + commits.size()); System.out.println(“Collected Pull Requests: ” + pullRequests.size()); System.out.println(“Collected Pull Requests: ” + branchEvents.size()); List result = new ArrayList(); result.addAll(commits); result.addAll(pullRequests); result.addAll(branchEvents); TimeSeries ts = store.putTimeSeries(“vcsData”, VcsActivityDTO.class); for (VcsActivityDTO activity : result) { ts.append(activity); } } private HttpHeaders createHeaders() { HttpHeaders headers = new HttpHeaders(); headers.set(“Authorization”, “Bearer ” + githubApiToken); return headers; } // Method to retrieve commits from a specific repository public List getCommits(String repoOwner, String repoName) throws ParseException { String url = UriComponentsBuilder.fromHttpUrl(githubBaseUrl) .path(“/repos/{owner}/{repo}/commits”) .buildAndExpand(repoOwner, repoName) .toUriString(); HttpHeaders headers = createHeaders(); HttpEntity entity = new HttpEntity(headers); ResponseEntity response = restTemplate.exchange(url, HttpMethod.GET, entity, List.class); List commitActivities = new ArrayList(); List commits = (List) response.getBody(); if (commits != null) { for (Map commit : commits) { commitActivities.add(mapCommitToVcsActivityDTO(commit)); } } return commitActivities; } public List getPullRequests(String repoOwner, String repoName) throws ParseException { String url = UriComponentsBuilder.fromHttpUrl(githubBaseUrl) .path(“/repos/{owner}/{repo}/pulls”) .queryParam(“state”, “all”) // Retrieves both open and closed pull requests .buildAndExpand(repoOwner, repoName) .toUriString(); HttpHeaders headers = createHeaders(); HttpEntity entity = new HttpEntity(headers); ResponseEntity response = restTemplate.exchange(url, HttpMethod.GET, entity, List.class); List prActivities = new ArrayList(); List pullRequests = (List) response.getBody(); if (pullRequests != null) { for (Map pr : pullRequests) { prActivities.add(mapPullRequestToVcsActivityDTO(pr)); } } return prActivities; } private VcsActivityDTO mapCommitToVcsActivityDTO(Map commitData) throws ParseException { Map commitInfo = (Map) commitData.get(“commit”); Map authorInfo = (Map) commitInfo.get(“author”); String eventType = “Commit”; String developerId = (String) authorInfo.get(“name”); String repositoryId = (String) commitInfo.get(“url”); String branch = “main”; String status = “Success”; String timestamp = (String) authorInfo.get(“date”); return new VcsActivityDTO(new SimpleDateFormat(“yyyy-MM-dd’T’HH:mm:ss’Z'”).parse(timestamp), eventType, developerId, repositoryId, branch, status); } private VcsActivityDTO mapPullRequestToVcsActivityDTO(Map prData) throws ParseException { String eventType = “Pull Request”; Map userInfo = (Map) prData.get(“user”); String developerId = (String) userInfo.get(“login”); String repositoryId = (String) userInfo.get(“repos_url”);; String branch = (String) ((Map)prData.get(“base”)).get(“ref”); String status = (String) prData.get(“state”); String timestamp = (String) prData.get(“created_at”); return new VcsActivityDTO(new SimpleDateFormat(“yyyy-MM-dd’T’HH:mm:ss’Z'”).parse(timestamp), eventType, developerId, repositoryId, branch, status); } public List getBranchEvents(String repoOwner, String repoName) throws ParseException{ String url = UriComponentsBuilder.fromHttpUrl(githubBaseUrl) .path(“/repos/{owner}/{repo}/events”) .buildAndExpand(repoOwner, repoName) .toUriString(); HttpHeaders headers = createHeaders(); HttpEntity entity = new HttpEntity(headers); ResponseEntity response = restTemplate.exchange(url, HttpMethod.GET, entity, List.class); List branchActivities = new ArrayList(); List events = response.getBody(); if (events != null) { for (Map eventData : events) { String eventType = (String) eventData.get(“type”); if (“CreateEvent”.equals(eventType) || “DeleteEvent”.equals(eventType)) { Map payload = (Map) eventData.get(“payload”); String refType = (String) payload.get(“ref_type”); if (“branch”.equals(refType)) { String branchName = (String) payload.get(“ref”); String developerId = (String) ((Map)eventData.get(“actor”)).get(“login”); String timestamp = (String) eventData.get(“created_at”); // Map to VcsActivityDTO VcsActivityDTO activity = new VcsActivityDTO(new SimpleDateFormat(“yyyy-MM-dd’T’HH:mm:ss’Z'”).parse(timestamp), eventType.equals(“CreateEvent”) ? “Branch Creation” : “Branch Deletion”, developerId, repoName, branchName, “Success” ); branchActivities.add(activity); } } } } return branchActivities; } } By following above steps, we can effectively extract data on developer activities from github, load it into GridDB. Data Querying in GridDB and Visualization with Thymeleaf Once the data is stored and available in GridDB, the next step is to visualize this data in a way that provides actionable insights. In this section, we’ll explore how to build a dashboard using Spring Boot, Thymeleaf, and Chart.js to render charts that display commits and pull request trends over time. Here are the steps to achieve this: Building the Chart Controller The ChartController acts as the intermediary between backend data in GridDB and the frontend visualizations displayed on the dashboard. Its responsibilities include handling HTTP requests, interacting with the service layer to fetch data, and passing that data to Thymeleaf templates for rendering. Here’s how the ChartController is implemented: package mycode.controller; import org.springframework.beans.factory.annotation.Autowired; import org.springframework.stereotype.Controller; import org.springframework.ui.Model; import org.springframework.web.bind.annotation.GetMapping; import com.fasterxml.jackson.databind.ObjectMapper; import mycode.service.ChartService; import mycode.dto.VcsActivityDTO; import java.text.SimpleDateFormat; import java.util.HashMap; import java.util.List; import java.util.Map; @Controller public class ChartController { @Autowired ChartService chartService; @Autowired private ObjectMapper objectMapper; @GetMapping(“/charts”) public String showCharts(Model model) { try { List events = chartService.getVcsEvents(); model.addAttribute(“events”, events); // Prepare data for charts Map commitData = prepareCommitData(events); Map prData = preparePullRequestData(events); // Convert Maps to JSON Strings for use in JavaScript in the Thymeleaf template String commitDataJson = objectMapper.writeValueAsString(commitData); String prDataJson = objectMapper.writeValueAsString(prData); model.addAttribute(“commitDataJson”, commitDataJson); model.addAttribute(“prDataJson”, prDataJson); } catch (Exception e) { e.printStackTrace(); } return “charts”; } private Map prepareCommitData(List events) { Map commitMap = new HashMap(); SimpleDateFormat dateFormat = new SimpleDateFormat(“yyyy-MM-dd”); for (VcsActivityDTO event : events) { if (“Commit”.equals(event.getEventType())) { String timestamp = dateFormat.format(event.getTimestamp()); commitMap.put(timestamp, commitMap.getOrDefault(timestamp, 0) + 1); } } return commitMap; } private Map preparePullRequestData(List events) { Map prMap = new HashMap(); SimpleDateFormat dateFormat = new SimpleDateFormat(“yyyy-MM-dd”); // Date format for (VcsActivityDTO event : events) { if (“Pull Request”.equals(event.getEventType())) { String timestamp = dateFormat.format(event.getTimestamp()); prMap.put(timestamp, prMap.getOrDefault(timestamp, 0) + 1); } } return prMap; } } Implementing the Chart Service The ChartService acts as the business logic layer, encapsulating the operations needed to query GridDB and process the results. The ChartService class retrieves VCS (Version Control System) events from a GridStore database container called “vcsData”. The service processes each row of the result, mapping fields like Timestamp, EventType, DeveloperId, RepositoryId, Branch, and Status into a VcsActivityDTO object. Finally, it returns a list of these DTOs representing the VCS activity events. Here’s how the ChartService is implemented: package mycode.service; import java.text.SimpleDateFormat; import java.util.ArrayList; import java.util.Date; import java.util.HashMap; import java.util.Map; import java.util.List; import org.springframework.beans.factory.annotation.Autowired; import org.springframework.stereotype.Service; import com.toshiba.mwcloud.gs.Container; import com.toshiba.mwcloud.gs.GridStore; import com.toshiba.mwcloud.gs.Query; import com.toshiba.mwcloud.gs.Row; import com.toshiba.mwcloud.gs.RowSet; import mycode.dto.VcsActivityDTO; @Service public class ChartService { @Autowired GridStore store; public List getVcsEvents() throws Exception {
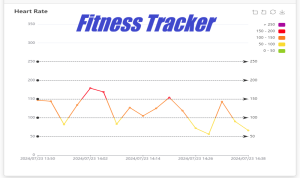
Being healthy and fit should be part of our lifestyle. Living a healthy lifestyle can help prevent long-term illnesses. One way to achieve that is by keeping records of our workouts, including the exercise type, duration, and heart rates. In this technical article, we’ll explore the process of creating a simple Fitness Tracking web using Spring Boot. We’ll start by creating a web app that can record our workouts – the when, what, and how long. We’re also going to tap into heart rate data and turn that data into a sleek line chart that shows our heart rate over time. It’s like giving your workout a visual heartbeat! We’ll break everything down into bite-sized pieces, explaining each step along the way. Features and Functionalities Here are some features that this web application will support: User can record their workout information like distance, calories, and start and end time. User can view the heart rate data in a line chart User can view the analysis of their heart rate zone In this article, we don’t provide: * RESTful endpoints to be consumed by mobile applications. * User registration. For demo purposes, we will provide demo users. * Integration with popular fitness APIs (Fitbit, Google Fit) * Specific metrics for each workout type The Data Model The complete data model. We’ll have a table users to store the details of people who use the application. For now, we don’t have user registration, so the user’s tables contain only name and email. Next, we’re talking about the fitness data storage. The main table is workouts which captures details of the workouts users perform. id – Assigns a unique ID to each workout. startTime and endTime – Hold the value of time when the workout starts and finished. distance – Sports like running or cycling are measured in terms of distance covered. calories – Hold the value of calories burnt by the user while doing a workout. We don’t use any formula to calculate the calories burnt, the user can input any value. duration – Auto populates by the system. To record the heart rate we create one table named heartrate. The columns in this table are: id – Assigns a unique ID to each heart rate data. workout_id – Identify which workout this data belongs to. value – Stores the heart rate value. time – Store the date and time when the heart rate value changed. To make the demo easier, we won’t have an option to enter heart rate values manually. Instead, we’ll create a function that automatically generates a random heart rate value every 3 minutes during the workout. Technology Stack Spring Boot: Open-source Java framework used for programming standalone, production-grade Spring-based applications with minimal effort. Spring Boot is a convention-over-configuration extension for the Spring Java platform intended to help minimize configuration concerns while creating Spring-based applications GridDB: Next-generation open-source database that makes time series IoT and big data fast, and easy. Thymeleaf: Java XML/XHTML/HTML5 template engine that can work both in web and non-web environments. It is better suited for serving XHTML/HTML5 at the view layer of MVC-based web applications, but it can process any XML file even in offline environments. It provides full Spring Framework integration. Apache EChart: A declarative framework for rapid construction of Web-based visualization. Apache ECharts provides more than 20 chart types available out of the box, along with a dozen components, and each of them can be arbitrarily combined to use. Maven: Build automation tool used primarily for Java projects. Docker: is an open source platform that enables developers to build, deploy, run, update and manage containerized applications Setting Up the Development Environment First, we need to install Java 17 or later, Maven 3.5+, Docker engine, and text editor (Intellij IDEA, or VSCode). To start from scratch, visit Spring Initializr and add dependencies Spring Web, Thymeleaf, Lombok, and Spring Boot DevTools. After that, click on the generate button and download the zip file. Unzip the file and open the project in your favorite IDE. Next, we should add the required dependencies. For interacting with GridDB, we need to add a GridDB Java Client dependency like this: <dependency> <groupId>com.github.griddb</groupId> <artifactId>gridstore</artifactId> <version>5.5.0</version> </dependency> The complete pom.xml file can be found here. Key Feature Implementation In this project, we will use the Controller-Service-Repository (CSR) pattern. This pattern promotes the separation of concern by dividing the application logic into three distinct layers: the Controller, the Service, and the Repository. Each layer has a specific responsibility, making the code easier to manage. After completing the Maven dependencies, we need to create several folders as follows: src/main/java/com/galapea/techblog/fitnesstracking/ ├── config ├── controller ├── entity ├── model └── service To be able to store the fitness information in GridDB database, we need to define the entity classes inside the entity folder. Create a new domain object to present a User as the following example: @Data public class User { @RowKey String id; String email; String fullName; Date createdAt; } Workout @Builder @NoArgsConstructor @AllArgsConstructor public class Workout { @RowKey public String id; public String title; public String type; public String userId; public Date startTime; public Date endTime; public Double distance; public Double duration; public Double calories; public Date createdAt; } HeartRate @Data public class HeartRate { @RowKey String id; Date timestamp; double value; String workoutId; } After that, we need to configure the database connection. Since we don’t have the Spring Boot auto-configuration for GridDB, so we will configure them by creating a GridDBConfig. We used the entity classes created earlier in this config to represent the container (table) in the database. @Configuration public class GridDBConfig { @Value(“${GRIDDB_NOTIFICATION_MEMBER}”) private String notificationMember; @Value(“${GRIDDB_CLUSTER_NAME}”) private String clusterName; @Value(“${GRIDDB_USER}”) private String user; @Value(“${GRIDDB_PASSWORD}”) private String password; @Bean public GridStore gridStore() throws GSException { Properties properties = new Properties(); properties.setProperty(“notificationMember”, notificationMember); properties.setProperty(“clusterName”, clusterName); properties.setProperty(“user”, user); properties.setProperty(“password”, password); GridStore store = GridStoreFactory.getInstance().getGridStore(properties); return store; } @Bean public Collection userCollection(GridStore gridStore) throws GSException { Collection collection = gridStore.putCollection(AppConstant.USERS_CONTAINER, User.class); collection.createIndex(“email”); return collection; } @Bean public Collection heartRateCollection(GridStore gridStore) throws GSException { Collection heartRateCollection = gridStore.putCollection(AppConstant.HEARTRATE_CONTAINER, HeartRate.class); heartRateCollection.createIndex(“workoutId”); return heartRateCollection; } @Bean public Collection workoutCollection(GridStore gridStore) throws GSException { Collection workoutCollection = gridStore.putCollection(AppConstant.WORKOUT_CONTAINER, Workout.class); workoutCollection.createIndex(“userId”); workoutCollection.createIndex(“title”); workoutCollection.createIndex(“type”); return workoutCollection; } } To access GridDB, we need to get a GridStore instance using the GridStoreFactory. Connecting to GridDB cluster using the fixed list method, we need to specify the following required properties: notificationMember A list of address and port pairs in cluster. It is used to connect to cluster which is configured with FIXED_LIST mode, and specified as follows. (Address1):(Port1),(Address2):(Port2),… This property cannot be specified with neither notificationAddress nor notificationProvider properties at the same time. This property is supported on version 2.9 or later. clusterName A cluster name. It is used to verify whether it matches the cluster name assigned to the destination cluster. If it is omitted or an empty string is specified, cluster name verification is not performed. Listing workout We create an HTML template workouts.html to display the list of workouts using the th:each dialect: <tbody class=”table-group-divider”> <tr th:each=”workout : ${workouts}”> <td id=”user” scope=”row” th:text=”${workout.user} ? ${workout.user.fullName} : ””></td> <th id=”type” scope=”row”>[[${workout.type}]]</th> <td> <a class=”small” th:href=”@{/heartrate/{id}(id=${workout.id})}” th:text=”${workout.title}”></a> </td> <th id=”distance” scope=”row”>[[${workout.distance}]]</th> <td id=”startTime” scope=”row”>[[${workout.startTime}]]</td> <th id=”endTime” scope=”row”>[[${workout.endTime}]]</th> <th id=”duration” scope=”row”>[[${workout.durationText}]]</th> </tr> </tbody> That template will be called by a controller class WorkoutController: @Controller @RequestMapping(“/workouts”) @RequiredArgsConstructor public class WorkoutController { private final WorkoutService workoutService; private final UserService userService; java.text.SimpleDateFormat formatter = new java.text.SimpleDateFormat(“yyyy-MM-dd’T’HH:mm”); @GetMapping String workouts(Model model) { List workouts = workoutService.fetchAll(); workouts.forEach(workout -> { try { workout.setUser(userService.fetchOneById(workout.getUserId())); } catch (GSException e) { e.printStackTrace(); } String durationText = Helper.getDurationText(workout.getStartTime(), workout.getEndTime()); workout.setDurationText(durationText); }); model.addAttribute(“workouts”, workouts); model.addAttribute(“createWorkout”, new CreateWorkout()); return “workouts”; } In the method workouts() we call fetchAll() method from WorkoutService class. And then before returning to the user interface, we need to get the full name of the user and format the duration into the human-readable format, for example: 1h 15m instead of the default java.util.Date format. We return a new model to the view to prepare for creating a new workout.\n Next, we need the service class to fetch data from GridDB. Here we create a query object to execute TQL statement for selecting all the records from the workout collection. And we mapped the object into the WorkoutDto. public List fetchAll() { List dtos = new ArrayList(0); try { String tql = “SELECT * FROM ” + AppConstant.WORKOUT_CONTAINER + ” ORDER BY createdAt DESC”; Query query = workoutCollection.query(tql); RowSet rowSet = query.fetch(); while (rowSet.hasNext()) { Workout row = rowSet.next(); WorkoutDto dto = WorkoutDto.builder() .id(row.id) .title(row.title) .type(WorkoutType.valueOf(row.type)) .userId(row.userId) .startTime(row.startTime) .endTime(row.endTime) .distance(row.distance) .duration(row.duration) .calories(row.calories) .createdAt(row.createdAt) .build(); dtos.add(dto); } } catch (GSException e) { log.error(“Error fetch all workouts”, e); } return dtos; } Record a workout When the user records a new workout and clicks submit, then we handle the form submission in the WorkoutController with the following method: @PostMapping(“/record”) String recordWorkout(@ModelAttribute(“createWorkout”) CreateWorkout createWorkout, final BindingResult bindingResult, RedirectAttributes attributes, Model model) throws ParseException { List users = userService.fetchAll(); java.util.Collections.shuffle(users); java.util.Date startDate = formatter.parse(createWorkout.getStartTime()); java.util.Date endDate = formatter.parse(createWorkout.getEndTime()); LocalDateTime start = Helper.convertToLocalDateTime(startDate); LocalDateTime end = Helper.convertToLocalDateTime(endDate); if (end.isBefore(start)) { bindingResult.rejectValue(“endTime”, “error.endTime”, “End-date must be after Start-date!”); attributes.addAttribute(“hasErrors”, true); } if (bindingResult.hasErrors()) { return workouts(model); } WorkoutDto workoutDto = WorkoutDto.builder() .title(createWorkout.getTitle()) .type(WorkoutType.valueOf(createWorkout.getType())) .userId(users.get(0).getId()) .distance(Double.parseDouble(createWorkout.getDistance())) .startTime(startDate) .endTime(endDate) .calories(Double.parseDouble(createWorkout.getCalories())) .build(); workoutService.create(workoutDto); attributes.addFlashAttribute(“message”, “Workout recorded!”); return “redirect:/workouts”; } For demo purposes, we select users randomly from the existing users. And then the controller will call the create method of WorkoutService. In this method, we calculate the duration of the workout. After inserting the row, we publish a WorkoutCreated event using ApplicationEventPublisher to be consumed by HeartRateService. public void create(WorkoutDto workoutDto) { try { Double duration = workoutDto.getDuration() == null ? 0.0 : workoutDto.getDuration(); if (workoutDto.getId() == null) { workoutDto.setId(KeyGenerator.next(“wk_”)); } Workout workout = Workout.builder() .id(workoutDto.getId()) .title(workoutDto.getTitle()) .type(workoutDto.getType().name()) .userId(workoutDto.getUserId()) .startTime(workoutDto.getStartTime()) .endTime(workoutDto.getEndTime()) .distance(workoutDto.getDistance()) .duration(duration) .calories(workoutDto.getCalories()) .createdAt(new java.util.Date()) .build(); if (workoutCollection.get(workoutDto.getId()) == null) { workoutCollection.put(workoutDto.getId(), workout); applicationEventPublisher.publishEvent(mapStructMapper.workoutToWorkoutCreated(workout)); } } catch (GSException e) { log.error(“Error create a workout”, e); } } If you notice, we said in the beginning that we will also record the heart rate data, but we don’t see any interface to input it. In this tutorial, we don’t connect with the wearable devices, so we decided to create a dummy heart rate data for each workout created. The logic is to create a random heart rate value every 3 minutes of the workout durations as shown in the following HeartRateService class. public void generateHeartRate(WorkoutCreated workout) { Faker faker = new Faker(); LocalDateTime start = Helper.convertToLocalDateTime(workout.getStartTime()); LocalDateTime end = Helper.convertToLocalDateTime(workout.getEndTime()); for (LocalDateTime date = start; date.isBefore(end); date = date.plusMinutes(3)) { HeartRate heartRate = new HeartRate(); heartRate.setWorkoutId(workout.getId()); heartRate.setValue(faker.number().randomDouble(0, 50, 190)); heartRate.setTimestamp(Date.from(date.toInstant(ZoneOffset.UTC))); heartRate.setId(KeyGenerator.next(“hr_”)); try { heartRateCollection.put(heartRate); log.debug(“Append: {}”, heartRate); } catch (GSException e) { log.error(“Error generateHeartRate”, e); } } } Generate dummy workout Before moving into the visualization, for the demo purpose, we will provide a method to generate a dummy workout. On creating dummy data we will utilize Java Faker a library that can be used to generate a wide array of real-looking data from addresses to popular culture references. @PostMapping(“/generate-dummy”) String generateDummyWorkout(RedirectAttributes attributes) { List users = userService.fetchAll(); List types = Arrays.asList(WorkoutType.values()); Faker faker = new Faker(); for (int i = 0; i < 2; i++) { LocalDateTime startDateTime = LocalDateTime.now().minusMinutes(faker.number().randomNumber(2, true)); LocalDateTime endDateTime = LocalDateTime.now().minusMinutes(faker.number().numberBetween(1, 3)); if (endDateTime.isBefore(startDateTime)) { continue; } Collections.shuffle(users); Collections.shuffle(types); WorkoutDto workoutDto = WorkoutDto.builder() .id(KeyGenerator.next("wk_")) .title(faker.weather().description() + " - " + faker.address().streetAddress() + ", " + faker.address().cityName()) .type(types.get(0)) .userId(users.get(0).getId()) .distance(Helper.calculateAverageDistanceInKm(startDateTime, endDateTime)) .calories(faker.number().randomDouble(0, 50, 500)) .startTime(Date.from(startDateTime.toInstant(ZoneOffset.UTC))) .endTime(Date.from(endDateTime.toInstant(ZoneOffset.UTC))) .build(); workoutService.create(workoutDto); } attributes.addFlashAttribute("message", "Generated dummy workout!"); return "redirect:/workouts"; } Heart Rate Visualization and Analytics Now we arrive at the visualization part.\ The first part is visualizing the heart rate over time for the selected workout. We use Apache EChart to create the line chart. Include EChart library from a CDN <script src="https://cdn.jsdelivr.net/npm/echarts@5.5.1/dist/echarts.min.js" integrity="sha256-6EJwvQzVvfYP78JtAMKjkcsugfTSanqe4WGFpUdzo88=" crossorigin="anonymous"></script> Create a DOM element to hold the chart <div class="chart-area"> <div id="heartrateChart" style="height:600px;"></div> </div> Creates an EChart instance, tied to the DOM container. Defines title, the x-axis using type category, the y-axis a value for numerical data, and defines series representing a dataset. var myChart = echarts.init(document.getElementById('heartrateChart')); const heartRateZoneSummaries = /*[[${heartRateZoneSummaries}]]*/[] const _data = /*[[${heartRates}]]*/[ { timestampLabel: "2024/01/01 04:18", value: 10 }, { timestampLabel: "2024/01/02 04:23", value: 5 } ]; var option = { title: { text: 'Heart Rate', left: '1%' }, tooltip: { trigger: 'axis' }, grid: { left: '10%', right: '15%', bottom: '10%' }, xAxis: { type: 'category', boundaryGap: false, data: _data.map(x => x.timestampLabel) }, yAxis: { type: ‘value’, boundaryGap: [0, ‘100%’] }, toolbox: { right: 10, feature: { dataZoom: { yAxisIndex: ‘none’ }, restore: {}, saveAsImage: {} } }, series: [ { name: ‘Heart Rate’, type: ‘line’, data: _data.map(x => x.value), markLine: { silent: true, lineStyle: { color: ‘#333’ }, data: [ { yAxis: 50 }, { yAxis: 100 }, { yAxis: 150 }, { yAxis: 200 }, { yAxis: 250 } ] } } ], }; // Display the chart using the configuration items and data just specified. myChart.setOption(option); After defining the chart object in the HTML, we need to provide the heart rata data from the controller class.\ HeartRateController: @GetMapping(“/{id}”) String heartRate(Model model, @PathVariable(“id”) String id) { log.info(“Fetch heart rate of workout: {}”, id); HeartRateDashboard heartRateDashboard = heartRateService.fetchForDashboardByWorkoutId(id); log.info(“heart rate size: {}”, heartRateDashboard.heartRates().size()); model.addAttribute(“heartRates”, heartRateDashboard.heartRates()); model.addAttribute(“workout”, workoutService.fetchById(id)); model.addAttribute(“heartRateZoneSummaries”, heartRateDashboard.heartRateZoneSummaries()); return “heartrate”; } The controller will supply the list of heart rates and zona summary into the view. Here are the DTO classes: public record HeartRateDashboard(List heartRates, List heartRateZoneSummaries) {} @Data public class HeartRateDto { private String timestampLabel; private double value; } @Data @Builder public class HeartRateZoneSummary { String zoneValue; long durationInMinuets; } In the service class, we query the heart rate container by workout ID and then format the timestamp into our desired format “2024/01/01 04:18”. Besides that, we categorize the heart rate data into 3 zones which are the Peak Zone, Cardio Zone, and Fat Burn Zone. HeartRateService: public HeartRateDashboard fetchForDashboardByWorkoutId(String workoutId) { List heartRates = new ArrayList(0); List heartRatesList = getHeartRatesList(workoutId); heartRates = heartRatesList.stream() .map(hr -> HeartRateDto.builder() .timestampLabel(getTimestampLabel(hr.getTimestamp())) .value(hr.getValue()) .build()) .collect(Collectors.toList()); List heartRateZoneSummaries = HeartRateAnalyzer.analyze(heartRatesList); return new HeartRateDashboard(heartRates, heartRateZoneSummaries); } private List getHeartRatesList(String workoutId) { List heartRatesList = new ArrayList(0); try { Query query = heartRateCollection.query(“SELECT * WHERE workoutId='” + workoutId + “‘”, HeartRate.class); RowSet rowSet = query.fetch(); while (rowSet.hasNext()) { HeartRate row = rowSet.next(); log.debug(“Fetched: {}”, row); heartRatesList.add(row); } } catch (GSException e) { log.error(“Error query heart rate”, e); } return heartRatesList; } Finally here is the dasboard: We use Docker Compose for defining and running this multi-container Docker application (Spring Boot application and GridDB). To run the project, please follow the instructions on Github. Conclusion In this tutorial, we have explored the power of Spring Boot in creating a robust MVC application. We showcase how to integrate GridDB as a data persistence. The integration of front-end technologies like Thymeleaf demonstrated the flexibility of Spring Boot in accommodating various UI templating. We’ve learned how to transform raw fitness data into meaningful visualizations. We can enhance this application with the following features: * Implement paginations on listing workouts * Provide API that can be used by wearable devices or pull workout details from the fitness API
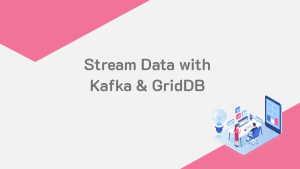
In today’s article we will be discussing Kafka in conjunction with GridDB, which we have done before: Stream Data with GridDB and Kafka Using GridDB as a source for Kafka with JDBC Using SQL Batch Inserts with GridDB v5.5, JDBC, and Kafka Udemy course: Create a working IoT Project – Apache Kafka, Python, GridDB We will focus in this article on a new feature which allows for use of Kafka with GridDB as a sink resource which will make TIME_SERIES containers (meaning we can push time_series data from Kafka topics directly into GridDB with some configuration); prior to v5.6, we were limited to Collection Containers. There will be some similarities with the blog last written about using Kafka with GridDB titled: “Stream Data with GridDB and Kafka”. The differences here are that we have made all the moving parts of kafka and GridDB into Docker containers for easier portability and ease of use and will, as alluded to earlier, be using Time Series containers. If you follow along with this blog, you will learn how use Kafka to stream time series data directly into a GridDB time series container using Docker containers and Kafka. High Level Overview Before we get into how to run this project, let’s briefly go over what this project does and how it works. We will get Kafka and GridDB running inside of docker containers, and once those are ready, we will run a python script which acts as a kafka producer to push up random data to the broker. This simulated iot data will then sit in a Kafka queue (though it’s more accurately a distributed log) until a consumer is available to read those values. In our case, GridDB will act as the sink, meaning it will consume the data topics made by our python script and then save that data into tables which will created by Kafka based on our topics’ schemas set within our Python script. To properly communicate how and where to save the Kafka topics, we will need to set up a GridDB Kafka Sink properties file. But first, we will also need to grab and build the latest version (v5.6) of the GridDB Kafka Connect and somehow share that with our running Kafka installation so that we may save time series data directly into time series containers. Within that properties file, we will need to set the container type to time_series along with various other important details. Getting Started Let’s discuss how to run this project. Prerequisites To follow along with this blog, you will need docker and docker compose for running Kafka and GridDB. We will also need python3 installed to create data to be pushed into Kafka as topics (and then eventually saved into GridDB). We will also need to grab and build the GridDB Kafka Connect jar file. GridDB Kafka Connect (Optional) You can download the latest version here: griddb-kafka-connect. To build, make sure you have maven installed and run: $ mvn clean install The .jar file will be created inside of the target directory under the name: griddb-kafka-connector-0.6.jar. Note: The jar file is also included in the source code provided by this repo (in the next section). If you clone the repo and run this project via docker compose, you do not need to download/build the jar file yourself. Source Code You can find the source code in the griddbnet github page: $ git clone https://github.com/griddbnet/Blogs.git –branch 7_kafka_timeseries Running Project Once you have the source code and docker installed, you can simply run: $ docker compose pull && docker compose up -d And then once it’s done, you can start checking if the Kafka connector has the GridDB sink properties file in place by running the following: $ curl http://localhost:8083/connectors/ [“griddb-kafka-sink”] You can also take a look at the contents of the kafka-sink to see what it contains: $ curl http://localhost:8083/connectors/griddb-kafka-sink Once that’s done, you can run the python script, which acts as a kafka producer. $ python3 -m pip install kafka-python $ python3 scripts/producer.py GridDB Sink Properties In Kafka and other stream/event-driven architectures, the concept of sources and sinks mean to describe the direction of the flow of data. The sink is where data flows in, or where the data ends up — in this case, we want our data payloads to persist inside of GridDB as time series data inside of a time series container. And so we set the properties file as such: connector.class= com.github.griddb.kafka.connect.GriddbSinkConnector name= griddb-kafka-sink cluster.name= myCluster user= admin password= admin notification.member= griddb-server=10001 container.type= TIME_SERIES topics= meter_0,meter_1,meter_2,meter_3 transforms= TimestampConverter transforms.TimestampConverter.type= org.apache.kafka.connect.transforms.TimestampConverter$Value transforms.TimestampConverter.format= yyyy-MM-dd hh=mm=ss transforms.TimestampConverter.field= timestamp transforms.TimestampConverter.target.type= Timestamp As compared to our previous article, the main changes are the container.type designation and the transforms properties. The transforms properties tells our Kafka cluster which string value will be converted into timestamp, along with other useful information to help that process along. The other values are simply allowing for our broker to know where to send the data topics to, which is our GridDB docker container with a hostname of griddb-server. The topics are the name of the data topics and will also be the names of our GridDB time series containers. Python Producer Script There isn’t much to say here that you can’t get from simply reading the (simple) source code. The only thing I will add is that if you wished to docker-ize the docker container as well, you would change server location from localhost to broker:9092 #p=KafkaProducer(bootstrap_servers=[‘localhost:9092’]) p=KafkaProducer(bootstrap_servers=[‘broker:9092′]) One other thing to note is that though we are making time_series data containers with time_series data as the row key, you still need to set your payload data fields as type string (I teased this above when discussing the transform property in the sink section). “schema”: { “fields”: [ { “field”: “timestamp”, “optional”: False, “type”: “string” }, { “field”: “kwh”, “optional”: False, “type”: “double” }, { “field”: “temp”, “optional”: False, “type”: “double” } ], “name”: “iot”, “optional”: False, “type”: “struct” } The key here is that though the type is string, we must set the first field as our targeted timestamp type. And then in the sink for this dataset, we set the transforms.TimestampConverter.field as the name of our field we want to convert to type timestamp. With these things in place, Kafka and GridDB will create your tables with the set schema and the proper container type. Running Kafka in Docker Containers In our previous article about kafka, we simply ran kafka and GridDB on bare metal, meaning simply running the servers throught he CLI with commands. Though it worked well, it’s a bit confusing because you need 3-4 terminals open and need to remember to run things in sequence. For this article, we have prepared a docker compose file which allows you to run download and run everything with 2-3 commands! Confluent Docker Containers First, let’s discuss the docker images provided by Confluent, which is a company which provides support and tools pertaining to Kafka for your large corporation. Despite this though, they provide the docker images freely which we will use in our docker compose file. Essentially what docker compose does is allow us to create a set of “services” (AKA docker containers) which we can run in unison with a simple command, with rules set in which we can set which containers rely on others. For example, we can set the various kafka containers to rely on each other so that they start up in the correct sequence. We opted for this because as explained above, running Kafka is not an easy process — it has many different parts that need to run. For example, to run this seemingly simple project where we push data from python script –> kafka topics –> GridDb it takes 5 services in our Docker compose file. Docker Compose Services The following are all of the services. GridDB Kafka Zookeeper Kafka Broker Kafka Schema Registry Kafka-Connect And another service which we omited but we could include is a kafka data producer. The Kafka zookeeper can be thought of as the brains or the main component of kafka. The Broker is the service which handles the data topics and is often run with many different brokers for failsafes, etc; when we want to point our producer of data topics to Kafka, we point to the broker. The kafka schema registry enforces schemas to be used for your topics. In our case, it’s useful for deserialization of our JSON schema of our data payloads from our python producer. The Kafka Connect container is where we add our third party libraries for use with Kafka: GridDB Kafka connect jar and our GridDB sink properties file. The connect container is a bit unique in that we need to make sure that the container is up and running first and then we push to it a json file with the GridDB sink property instructions. The GridDB Kafka Connect jar file though we push to the file system during docker image start up. Docker Compose Instructions For GridDB there are no special instructions: we simply pull the image from griddbnet and then set some environment variables: griddb-server: image: ‘griddbnet/griddb:5.6.0’ container_name: griddb-server expose: – ‘10001’ – ‘10010’ – ‘10020’ – ‘10040’ – ‘20001’ – ‘41999’ environment: NOTIFICATION_MEMBER: 1 GRIDDB_CLUSTER_NAME: myCluster The zookeeper is in a similar boat: zookeeper: image: ‘confluentinc/cp-zookeeper:7.3.0’ container_name: zookeeper environment: ZOOKEEPER_CLIENT_PORT: 2181 ZOOKEEPER_TICK_TIME: 2000 The broker exposes port 9092 so that we can run our python producer script outside of the context of our docker compose network environment (we just point to localhost:9092). There are also more environment variables necessary for pointing to the zookeeper and other cluster rules broker: image: ‘confluentinc/cp-kafka:7.3.0’ container_name: broker ports: – ‘9092:9092’ depends_on: – zookeeper environment: KAFKA_BROKER_ID: 1 KAFKA_ZOOKEEPER_CONNECT: ‘zookeeper:2181’ KAFKA_LISTENER_SECURITY_PROTOCOL_MAP: ‘PLAINTEXT:PLAINTEXT,PLAINTEXT_INTERNAL:PLAINTEXT’ KAFKA_ADVERTISED_LISTENERS: ‘PLAINTEXT://broker:9092,PLAINTEXT_INTERNAL://broker:29092’ KAFKA_OFFSETS_TOPIC_REPLICATION_FACTOR: 1 KAFKA_TRANSACTION_STATE_LOG_MIN_ISR: 1 KAFKA_TRANSACTION_STATE_LOG_REPLICATION_FACTOR: 1 You will also notice that the broker, schema registry, kafka connect all “depend” on the zookeeper. It really makes clear to us who is charge of the entire operation. kafka-schema-registry: image: ‘confluentinc/cp-schema-registry:7.3.0’ hostname: kafka-schema-registry container_name: kafka-schema-registry ports: – ‘8082:8082’ environment: SCHEMA_REGISTRY_KAFKASTORE_BOOTSTRAP_SERVERS: ‘PLAINTEXT://broker:9092’ SCHEMA_REGISTRY_HOST_NAME: kafka-schema-registry SCHEMA_REGISTRY_LISTENERS: ‘http://0.0.0.0:8082’ depends_on: – zookeeper The kafka connect also grabs from the confluent docker hub and has tons of environment variables, but it also includes volumes with a shared filesystem with the host machine so that we can share our GridDB Kafka Connect jar file. And lastly, we have scipt at the very bottom of the service which allows us to wait until our kafka-connect HTTP endpoints are available. Once we get a 200 status code as a response, we can run our script which sends our GridDB-Sink properties file. kafka-connect: image: confluentinc/cp-kafka-connect:latest container_name: kafka-connect ports: – ‘8083:8083’ environment: CONNECT_BOOTSTRAP_SERVERS: ‘broker:9092’ CONNECT_REST_PORT: 8083 CONNECT_GROUP_ID: device CONNECT_CONFIG_STORAGE_TOPIC: device-config CONNECT_OFFSET_STORAGE_TOPIC: device-offsets CONNECT_STATUS_STORAGE_TOPIC: device-status CONNECT_KEY_CONVERTER: org.apache.kafka.connect.json.JsonConverter CONNECT_VALUE_CONVERTER: org.apache.kafka.connect.json.JsonConverter CONNECT_INTERNAL_KEY_CONVERTER: org.apache.kafka.connect.json.JsonConverter CONNECT_INTERNAL_VALUE_CONVERTER: org.apache.kafka.connect.json.JsonConverter CONNECT_KEY_CONVERTER_SCHEMAS_ENABLE: true CONNECT_VALUE_CONVERTER_SCHEMAS_ENABLE: true CONNECT_KEY_CONVERTER_SCHEMA_REGISTRY_URL: ‘http://kafka-schema-registry:8082’ CONNECT_VALUE_CONVERTER_SCHEMA_REGISTRY_URL: ‘http://kafka-schema-registry:8082’ CONNECT_REST_ADVERTISED_HOST_NAME: kafka-connect CONNECT_LOG4J_APPENDER_STDOUT_LAYOUT_CONVERSIONPATTERN: ‘[%d] %p %X{connector.context}%m (%c:%L)%n’ CONNECT_CONFIG_STORAGE_REPLICATION_FACTOR: ‘1’ CONNECT_OFFSET_STORAGE_REPLICATION_FACTOR: ‘1’ CONNECT_STATUS_STORAGE_REPLICATION_FACTOR: ‘1’ CONNECT_PLUGIN_PATH: >- /usr/share/java,/etc/kafka-connect/jars CLASSPATH: >- /usr/share/java,/etc/kafka-connect/jars volumes: – ‘./scripts:/scripts’ – ‘./kafka-connect/connectors:/etc/kafka-connect/jars/’ depends_on: – zookeeper – broker – kafka-schema-registry – griddb-server command: – bash – ‘-c’ – > /etc/confluent/docker/run & echo “Waiting for Kafka Connect to start listening on kafka-connect ” while [ $$(curl -s -o /dev/null -w %{http_code} http://kafka-connect:8083/connectors) -eq 000 ] ; do echo -e $$(date) ” Kafka Connect listener HTTP state: ” $$(curl -s -o /dev/null -w %{http_code} http://kafka-connect:8083/connectors) ” (waiting for 200)” sleep 5 done nc -vz kafka-connect 8083 echo -e “\n–\n+> Creating Kafka Connect GridDB sink” /scripts/create-griddb-sink.sh && /scripts/example-sink.sh sleep infinity This properties file will give explicit instructions to Kafka that when topics with certain names are received by the broker, it should push those out to the instructions in the properties file, which in this case are our GridDB container. Conclusion After you run the producer, you should be able to see all of your data inside of your docker griddb server through use of the GridDB CLI: $ docker exec -it griddb-server gs_sh. And with that, we have successfully pushed IoT-like sensor data through Kafka to a GridDB Time Series
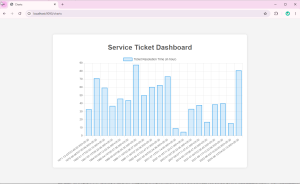
Introduction In today’s competitive landscape, efficient customer support is essential for retaining customers and driving business success. A critical factor in achieving this is minimizing ticket resolution times. By analyzing and optimizing these times, organizations can identify bottlenecks, improve support workflows, and enhance overall customer satisfaction Salesforce, a widely used CRM platform, provides a foundation for managing customer interactions. However, to gain deeper insights into ticket resolution times and identify areas for improvement, organizations can leverage the power of GridDB. This high-performance time series database is designed to handle large volumes of time-sensitive data efficiently. This blog will walk you through creating a dashboard that integrates Salesforce CRM service ticket data with GridDB for time series analysis and visualization of average ticket resolution times. We’ll cover how to extract data using RESTful APIs with Spring Boot, store it in GridDB, query the data for valuable insights, and ultimately visualize the results. Setting Up GridDB Cluster and Spring Boot Integration: For Real-Time Monitoring To effectively perform time series analysis on customer support ticket resolution times, the first step is to set up a GridDB cluster and integrate it with your Spring Boot application. Setting up GridDB Cluster GridDB provides flexible options to meet different requirements. For development, a single-node cluster on your local machine may be sufficient. However, in production, distributed clusters across multiple machines are typically preferred for improved fault tolerance and scalability. For detailed guidance on setting up clusters based on your deployment strategy, refer to the GridDB documentation. To set up a GridDB cluster, follow these steps mentioned here. Setting up Spring Boot Application Once your GridDB cluster is operational, the next step is connecting it to your Spring Boot application. The GridDB Java Client API provides the necessary tools to establish this connection. To simplify the process, you can include the griddb-spring-boot-starter library as a dependency in your project, which offers pre-configured beans for a streamlined connection setup. Project Structure Here’s a suggested project structure for such an application: my-griddb-app │ ├── pom.xml │ ├── src │ │ ├── main │ │ │ ├── java │ │ │ │ └── mycode │ │ │ │ ├── config │ │ │ │ │ └── GridDBConfig.java │ │ │ │ ├── controller │ │ │ │ │ └── ChartController.java │ │ │ │ ├── dto │ │ │ │ │ └── ServiceTicket.java │ │ │ │ ├── MySpringBootApplication.java │ │ │ │ └── service │ │ │ │ ├── ChartService.java │ │ │ │ ├── MetricsCollectionService.java │ │ │ │ └── RestTemplateConfig.java │ │ │ └── resources │ │ │ ├── application.properties │ │ │ └── templates │ │ │ └── charts.html This structure delineates distinct layers for controllers, models, repositories, services, and the application entry point, promoting modularity and maintainability. Additionally, it encompasses resource files such as application properties and logging configurations, alongside testing suites for ensuring robustness. Add GridDB Dependency To enable interaction with GridDB in your Spring Boot project, you must include the GridDB Java Client API dependency. This can be accomplished by adding the appropriate configuration to your project’s build file, such as pom.xml for Maven or the equivalent file for Gradle. Here’s an example of how to configure the dependency in your pom.xml file: <project xmlns=”http://maven.apache.org/POM/4.0.0″ xmlns:xsi=”http://www.w3.org/2001/XMLSchema-instance” xsi:schemaLocation=”http://maven.apache.org/POM/4.0.0 http://maven.apache.org/maven-v4_0_0.xsd”> <modelVersion>4.0.0</modelVersion> <groupId>com.example</groupId> <artifactId>my-griddb-app</artifactId> <version>1.0-SNAPSHOT</version> <name>my-griddb-app</name> <url>http://maven.apache.org</url> <parent> <groupId>org.springframework.boot</groupId> <artifactId>spring-boot-starter-parent</artifactId> <version>3.2.4</version> <relativePath /> <!– lookup parent from repository –> </parent> <properties> <maven.compiler.source>17</maven.compiler.source> <maven.compiler.target>17</maven.compiler.target> </properties> <dependencies> <dependency> <groupId>org.springframework.boot</groupId> <artifactId>spring-boot-starter-actuator</artifactId> </dependency> <dependency> <groupId>junit</groupId> <artifactId>junit</artifactId> <version>3.8.1</version> <scope>test</scope> </dependency> <!– GridDB dependencies –> <dependency> <groupId>com.github.griddb</groupId> <artifactId>gridstore-jdbc</artifactId> <version>5.3.0</version> </dependency> <dependency> <groupId>com.github.griddb</groupId> <artifactId>gridstore</artifactId> <version>5.5.0</version> </dependency> <!– Spring Boot dependencies –> <dependency> <groupId>org.springframework.boot</groupId> <artifactId>spring-boot-starter-web</artifactId> <exclusions> <exclusion> <groupId>org.springframework.boot</groupId> <artifactId>spring-boot-starter-logging</artifactId> </exclusion> </exclusions> </dependency> <dependency> <groupId>org.springframework.boot</groupId> <artifactId>spring-boot-starter-test</artifactId> <scope>test</scope> </dependency> <dependency> <groupId>org.springframework.boot</groupId> <artifactId>spring-boot-starter-thymeleaf</artifactId> </dependency> <!– JSON processing –> <dependency> <groupId>com.fasterxml.jackson.core</groupId> <artifactId>jackson-databind</artifactId> <version>2.15.0</version> <!– or the latest version –> </dependency> <!– Lombok –> <dependency> <groupId>org.projectlombok</groupId> <artifactId>lombok</artifactId> <optional>true</optional> </dependency> </dependencies> </project> Configure GridDB Connection After adding the GridDB dependency, the next step is to configure the connection details for your GridDB cluster in your Spring Boot application. This is typically done in the application.properties file, which is where you define various settings for your app. Here’s a quick example of how to set up those connection details: GRIDDB_NOTIFICATION_MEMBER=127.0.0.1:10001 GRIDDB_CLUSTER_NAME=myCluster GRIDDB_USER=admin GRIDDB_PASSWORD=admin management.endpoints.web.exposure.include=* server.port=9090 griddb.cluster.host: The hostname or IP address of your GridDB cluster. griddb.cluster.port: The port number on which the GridDB cluster is listening. griddb.cluster.user: The username for accessing the GridDB cluster. griddb.cluster.password: The password for the specified GridDB user (replace with your actual password). server.port=9090: Sets the port on which your Spring Boot application will run. Create GridDB Client Bean To interact with GridDB effectively in your Spring Boot application, you’ll need a dedicated Spring Bean to manage the GridDB connection. This bean will initialize the connection using the parameters specified in your application.properties file and will serve as the central point for interacting with the GridDB cluster throughout your application. Here’s an example of how to define this bean in a Java class named GridDbConfig.java: package mycode.config; import java.util.Properties; import org.springframework.beans.factory.annotation.Value; import org.springframework.context.annotation.Bean; import org.springframework.context.annotation.Configuration; import org.springframework.context.annotation.PropertySource; import com.toshiba.mwcloud.gs.GSException; import com.toshiba.mwcloud.gs.GridStore; import com.toshiba.mwcloud.gs.GridStoreFactory; @Configuration @PropertySource(“classpath:application.properties”) public class GridDBConfig { @Value(“${GRIDDB_NOTIFICATION_MEMBER}”) private String notificationMember; @Value(“${GRIDDB_CLUSTER_NAME}”) private String clusterName; @Value(“${GRIDDB_USER}”) private String user; @Value(“${GRIDDB_PASSWORD}”) private String password; @Bean public GridStore gridStore() throws GSException { // Acquiring a GridStore instance Properties properties = new Properties(); properties.setProperty(“notificationMember”, notificationMember); properties.setProperty(“clusterName”, clusterName); properties.setProperty(“user”, user); properties.setProperty(“password”, password); return GridStoreFactory.getInstance().getGridStore(properties); } } Metric Collection To visualize customer support ticket resolution times from Salesforce with GridDB, we first extract the necessary data using Salesforce’s REST API. Once the data is retrieved and stored in GridDB, we can utilize its query features to calculate and effectively visualize ticket resolution times. Here’s how to proceed with the data collection and loading process: Querying Salesforce Data Salesforce provides detailed information on customer support cases through its REST API, such as Id, CaseNumber, Subject, Status, CreatedDate, ClosedDate, and Priority. These fields are pivotal for monitoring ticket resolution times and evaluating your support team’s efficiency. To extract this data, we leverage Salesforce’s REST API, which allows us to perform queries using Salesforce Object Query Language (SOQL). The following steps outline the high-level process: Define the Query: Construct a SOQL query to select the relevant fields from the Case object. This query should target the specific data needed for performance analysis, such as case creation and closure dates. Authenticate and Send the Request: Utilize OAuth tokens to securely authenticate your application with Salesforce. Once authenticated, the query is sent to Salesforce’s API endpoint. Handle the Response: After receiving the API response, parse the returned JSON data to extract the required fields. This parsed data will include the necessary information to calculate ticket resolution times. Loading Data into GridDB Once we have retrieved the necessary data from Salesforce, the next step is to load it into GridDB. Here’s a high-level overview of this process: Data Transformation and Mapping: Convert Salesforce fields (such as CreatedDate, ClosedDate, Priority) to match the attributes in GridDB’s time series schema. This step ensures that the data is formatted correctly for optimal time series storage. We use the following DTO to define the GridDB schema. package mycode.dto; import lombok.AllArgsConstructor; import lombok.Data; import lombok.NoArgsConstructor; import java.util.Date; import com.toshiba.mwcloud.gs.RowKey; @Data @NoArgsConstructor @AllArgsConstructor public class ServiceTicket { @RowKey public Date createdDate; public String caseNumber; public Date closedDate; public String subject; public String status; public String priority; public double resolutionTime; } Insert Data into GridDB: Iterate over the transformed DTOs and insert each record into the corresponding GridDB container. Ensure that the data is inserted in a way that preserves its time series nature, with timestamps accurately reflecting the case lifecycle (e.g., CreatedDate and ClosedDate). The full implementation for this process is detailed in the following class. package mycode.service; import java.util.ArrayList; import java.util.Date; import java.util.Random; import java.text.ParseException; import java.time.Instant; import java.time.LocalDateTime; import java.time.ZoneOffset; import org.springframework.beans.factory.annotation.Autowired; import org.springframework.http.HttpEntity; import org.springframework.http.HttpHeaders; import org.springframework.http.HttpMethod; import org.springframework.http.HttpStatus; import org.springframework.http.MediaType; import org.springframework.http.ResponseEntity; import org.springframework.scheduling.annotation.Scheduled; import org.springframework.stereotype.Service; import org.springframework.util.LinkedMultiValueMap; import org.springframework.util.MultiValueMap; import org.springframework.web.client.HttpClientErrorException; import org.springframework.web.client.RestTemplate; import org.springframework.web.util.UriComponentsBuilder; import com.fasterxml.jackson.core.JsonProcessingException; import com.fasterxml.jackson.databind.JsonMappingException; import com.fasterxml.jackson.databind.JsonNode; import com.fasterxml.jackson.databind.ObjectMapper; import com.fasterxml.jackson.databind.node.ArrayNode; import com.toshiba.mwcloud.gs.*; import mycode.dto.ServiceTicket; @Service public class MetricsCollectionService { @Autowired private GridStore store; @Autowired private RestTemplate restTemplate; @Scheduled(fixedRate = 60000) // Collect metrics every minute public void collectMetrics() throws GSException, JsonMappingException, JsonProcessingException, ParseException { String accessToken = getSalesforceAccessToken(); ArrayList salesforceData = fetchSalesforceData(accessToken); salesforceData.forEach(ticket -> { try { TimeSeries ts = store.putTimeSeries(“serviceTickets”, ServiceTicket.class); ts.put(salesforceData); } catch (GSException e) { e.printStackTrace(); } }); } public ArrayList fetchSalesforceData(String accessToken) throws JsonMappingException, JsonProcessingException, ParseException { String queryUrl = “https://.develop.my.salesforce.com/services/data/v57.0/query”; HttpHeaders headers = new HttpHeaders(); headers.setBearerAuth(accessToken); UriComponentsBuilder builder = UriComponentsBuilder.fromHttpUrl(queryUrl) .queryParam(“q”, “SELECT+Id,+CaseNumber,+Subject,+Status,+CreatedDate,+ClosedDate,+Priority+FROM+Case”); HttpEntity request = new HttpEntity(headers); ResponseEntity response = restTemplate.exchange(builder.toUriString(), HttpMethod.GET, request, String.class); if (response.getStatusCode() == HttpStatus.OK) { ObjectMapper objectMapper = new ObjectMapper(); JsonNode rootNode = objectMapper.readTree(response.getBody()); ArrayNode records = (ArrayNode) rootNode.path(“records”); System.out.println(response.getBody()); ArrayList serviceTickets = new ArrayList(); for (JsonNode record : records) { ServiceTicket ticket = new ServiceTicket(); String status = record.get(“Status”).asText(); ticket.setStatus(status); if (“Closed”.equals(status)) { ticket.setCaseNumber(record.get(“CaseNumber”).asText()); ticket.setCreatedDate(objectMapper.convertValue(record.get(“CreatedDate”),Date.class)); ticket.setClosedDate(objectMapper.convertValue(record.get(“ClosedDate”), Date.class)); ticket.setSubject(record.get(“Subject”).asText()); ticket.setPriority(record.get(“Priority”).asText()); ticket.setResolutionTime( calculateResolutionTimeInHours( objectMapper.convertValue(record.get(“CreatedDate”), Date.class), objectMapper.convertValue(record.get(“ClosedDate”), Date.class))); serviceTickets.add(ticket); } } return serviceTickets; } else { throw new RuntimeException(“Failed to fetch data from Salesforce”); } } public static double calculateResolutionTimeInHours(Date createdDate, Date closedDate) { long timeDifferenceMillis = closedDate.getTime() – createdDate.getTime(); return 1 + (100 – 1) * new Random().nextDouble(); } public String getSalesforceAccessToken() throws JsonMappingException, JsonProcessingException { String url = “https://login.salesforce.com/services/oauth2/token”; HttpHeaders headers = new HttpHeaders(); headers.setContentType(MediaType.APPLICATION_FORM_URLENCODED); MultiValueMap body = new LinkedMultiValueMap(); body.add(“grant_type”, “password”); body.add(“client_id”, “ENTER_CLIENT_ID”); body.add(“client_secret”, “ENTER_CLIENT_SECRET”); body.add(“password”, “ENTER_PASSOWRD”); body.add(“redirect_uri”, “ENTER_REDIRECT_URI”); body.add(“username”, “ENTER_USERNAME”); HttpEntity requestEntity = new HttpEntity(body, headers); try { ResponseEntity response = restTemplate.exchange(url, HttpMethod.POST, requestEntity, String.class); if (response.getStatusCode() == HttpStatus.OK) { ObjectMapper objectMapper = new ObjectMapper(); JsonNode jsonNode = objectMapper.readTree(response.getBody()); return jsonNode.get(“access_token”).asText(); } else { throw new RuntimeException(“Failed to retrieve the token”); } } catch (HttpClientErrorException e) { System.out.println(“HTTP Error: ” + e.getStatusCode()); System.out.println(“Response Body: ” + e.getResponseBodyAsString()); throw e; } } } By following above steps, we can effectively extract customer support case data from Salesforce, load it into GridDB. Data Querying in GridDB and Visualization with Thymeleaf Once the data is stored and available in GridDB, the next step is to visualize this data in a way that provides actionable insights. In this section, we’ll explore how to build a dashboard using Spring Boot, Thymeleaf, and Chart.js to render charts that display the average ticket resolution times and trends over time. Here are the steps to achieve this: Building the Chart Controller The ChartController acts as the intermediary between backend data in GridDB and the frontend visualizations displayed on the dashboard. Its responsibilities include handling HTTP requests, interacting with the service layer to fetch data, and passing that data to Thymeleaf templates for rendering. Here’s how the ChartController is implemented: package mycode.controller; import org.springframework.beans.factory.annotation.Autowired; import org.springframework.stereotype.Controller; import org.springframework.ui.Model; import org.springframework.web.bind.annotation.GetMapping; import mycode.service.ChartService; import java.util.HashMap; import java.util.Map; @Controller public class ChartController { @Autowired ChartService chartService; @GetMapping(“/charts”) public String showCharts(Model model) { Map chartData = new HashMap(); try { Map projectionData = chartService.queryData(); chartData.put(“values”, projectionData.get(“time”)); chartData.put(“labels”, projectionData.get(“dates”)); } catch (Exception e) { e.printStackTrace(); } model.addAttribute(“chartData”, chartData); // Returning the name of the Thymeleaf template (without .html extension) return “charts”; } } Implementing the Chart Service The ChartService acts as the business logic layer, encapsulating the operations needed to query GridDB and process the results. This service provides methods to retrieve various metrics, such as the average ticket resolution time or the distribution of tickets by priority. Here’s how the ChartService is implemented: package mycode.service; import java.text.SimpleDateFormat; import java.util.ArrayList; import java.util.Date; import java.util.HashMap; import java.util.Map; import org.springframework.beans.factory.annotation.Autowired; import org.springframework.stereotype.Service; import com.toshiba.mwcloud.gs.Container; import com.toshiba.mwcloud.gs.GridStore; import com.toshiba.mwcloud.gs.Query; import com.toshiba.mwcloud.gs.Row; import com.toshiba.mwcloud.gs.RowSet; @Service public class ChartService { @Autowired GridStore store; public Map queryData() throws Exception {
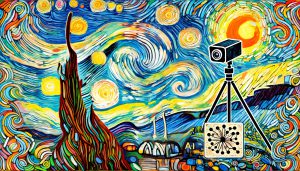
In this tutorial, we will explore how to extract color palettes from images captured via a webcam using Node.js, GridDB, and OpenAI. By leveraging Node.js for server-side scripting, GridDB for efficient data storage, and OpenAI for advanced image processing, we will create a seamless pipeline to capture images, analyze them, and generate dynamic color palettes. This guide will walk you through setting up your environment, capturing images from your webcam, and using AI to extract and store color data effectively. Prerequisites Before we dive in, ensure the following software is installed on your machine: Node.js GridDB OpenAI API access Browser with a webcam access Running The Project Clone the source code from this GitHub repository. git clone https://github.com/griddbnet/Blogs.git –branch color-extraction This project also needs to install Node.js and GridDB for this project to run. If the software requirements are installed, change the directory to the apps project directory and then install all the dependencies: cd color-detection-openai cd apps npm install Create a .env file and copy all environment variables from the .env.example file. We need an OpenAI key for this project, please look in the “Getting Started” section to get started. OPENAI_API_KEY=sk-proj-secret VITE_APP_URL=http://localhost:3000 You can change the VITE_APP_URL to your needs and then run the project by running this command: npm run start:build Go to the browser and enter the URL set on VITE_APP_URL, which in this case is http://localhost:3000. Make sure to enable the webcam in your browser, then click the Capture button to take a photo using the web camera. Setting Up the Environment 1. Installing Node.js This project will run on the Node.js platform. You need to install it from here. For this project, we will use the nvm package manager and Node.js v16.20.2 LTS version. # installs nvm (Node Version Manager) curl -o- https://raw.githubusercontent.com/nvm-sh/nvm/v0.39.7/install.sh | bash # download and install Node.js nvm install 16 # verifies the right Node.js version is in the environment node -v # should print `v16.20.2` # verifies the right NPM version is in the environment npm -v # should print `8.19.4“ To connect Node.js and GridDB database, we need the gridb-node-api npm package which is a Node.js binding developed using GridDB C Client and Node addon API. 2. Setting Up GridDB We will use the GridDB database to save recipes and it’s nutrition analysis. Please look at the guide for detailed installation. We will use Ubuntu 20.04 LTS here. Run GridDB and check if the service is running. Use this command: sudo systemctl status gridstore If not running try to run the database with this command: sudo systemctl start gridstore 3. Get The OpenAI Key To get the OpenAI key, create a project first and then create a key. The important thing is you should save the OpenAI key on the .env file and ensure not to include it in version control by adding it to the .gitignore. OPENAI_API_KEY=sk-proj-secret Another crucial factor is to select models that are accessible for the project. For this project, we will utilize gpt-4o models for image recognition and extracting colors from the image. The AI model’s response is non-deterministic, which means sometimes the response is not exactly what we want. By default this project uses the gpt-4o-mini model, in case the response is not quite right, you can change it to a more powerful model, such as the gpt-4o model. Capturing Images with MediaStream To capture images, we can use MediaStream API. It is an API related to WebRTC which provides support for streaming audio and video data. Before capturing an image from the web camera, we first need to initialize the web camera: const initializeWebcam = () => { navigator.mediaDevices.getUserMedia({ video: true }) .then(stream => { videoRef.current.srcObject = stream }) .catch(error => { console.error(‘getUserMedia error:’, error) }) } And then to capture the image from the video, we can use the drawImage() function: const captureImage = () => { const context = canvasRef.current.getContext(‘2d’) context.drawImage(videoRef.current, 0, 0, canvasRef.current.width, canvasRef.current.height) const base64Image = canvasRef.current.toDataURL(‘image/jpeg’) processImage(base64Image) } The drawImage() function will capture the current frame from the video stream and render it onto the canvas. This allows for further image data manipulation, processing, or conversion. In the provided code, the drawn image on the canvas is converted to a base64-encoded string using the toDataURL() function, which is then sent to a server for processing. Processing Images with OpenAI The image processing on the server is quite simple. The web app will send a base64-encoded image to the /process-image route. app.post(‘/process-image’, async (req, res) => { const { image } = req.body if (!image) { return res.status(400).json({ error: ‘No image provided’ }) } // eslint-disable-next-line no-undef const result = await getColorAnalysis(image) res.json(result.choices[0]) }) Then to get the color analysis from the image, we will use the gpt-4o-mini model from OpenAI. The getColorAnalysis() function will take the base64-encoded image and then process it. async function getColorAnalysis(base64Image) { const response = await openai.chat.completions.create({ model: “gpt-4o-mini-2024-07-18”, messages: [{ role: “system”, content: systemPrompt }, { role: “user”, content: [{ type: “image_url”, image_url: { url: base64Image } }, { type: “text”, text: userPrompt } ] } ], temperature: 0.51, max_tokens: 3000, top_p: 1, frequency_penalty: 0, presence_penalty: 0, }); return response; } OpenAI’s model response is determined by the prompt given. For a color analysis, use the specific prompt: const userPrompt = “Extract the seven most prominent colors from the provided image. Use color clustering techniques to identify and present these colors in Hex values. Answer with the raw array values ONLY. DO NOT FORMAT IT.”; We can get a better result by adding a system prompt to the OpenAI model. This system prompt behaves like a command for the OpenAI model to behave for a specific persona, which is a professional color analyst. const systemPrompt = `You are an AI specialized in colorimetry, the science and technology of color detection and measurement. You possess deep knowledge of the principles of color science, including color spaces, color matching functions, and the use of devices such as spectrophotometers and colorimeters. You provide accurate and detailed analyses of color properties, offer solutions for color consistency issues, and assist in applications ranging from imaging and printing to manufacturing and display technologies. Use your expertise to answer questions, solve problems, and provide color detection and measurement guidance.`; The prompt can also specify the model format response. In this project, we want the array of colors from the image colors analysis. The OpenAI model response should be in the form: [‘#2A2C9B’, ‘#F08A7D’, ‘#8E5DB2’, ‘#E8A1A3’, ‘#4D3B9E’, ‘#7F3C8F’, ‘#B57AB3’] Where each item in the array is a color in the hex format. Storing Data in GridDB We utilize the GridDB database for data storage. Here are the main data fields along with their descriptions: Column Name Type Description id INTEGER Unique identifier for each row. picture BLOB Base64 image encoding. colors STRING List of colors in Hex format. The saveData() function is a wrapper for the insert() function in the libs\griddb.cjs file. It is responsible for saving data into the database. Only two main fields are saved in the database. export async function saveData({ image, genColors }) { const id = generateRandomID() const picture = Buffer(image) const colors = String(genColors) const packetInfo = [parseInt(id), picture, colors] const saveStatus = await GridDB.insert(packetInfo, collectionDb) return saveStatus } The save data function will be executed on the server route /process-image after the color analysis of the image. Every time a user captures an image, it will be automatically sent to the server and the resulting data will be saved to the database. app.post(‘/process-image’, async (req, res) => { const { image } = req.body if (!image) { return res.status(400).json({ error: ‘No image provided’ }) } // eslint-disable-next-line no-undef const result = await getColorAnalysis(image) const colorsArray = result.choices[0].message.content // save data to the database const saveStatus = await saveData(image, colorsArray) console.log(saveStatus) res.json(result.choices[0]) }) Building User Interfaces The UI comprises two primary user interfaces: image capture and color palettes. React.js is utilized in this project to improve component management. Image Capture The image capture user interface is simply an HTML5 video view. This is the snippet code that shows the main HTML tags used: // WebcamContainer.js const WebcamContainer = ({ onColorsExtracted }) => { const captureImage = () => { const context = canvasRef.current.getContext(‘2d’) context.drawImage(videoRef.current, 0, 0, canvasRef.current.width, canvasRef.current.height) const base64Image = canvasRef.current.toDataURL(‘image/jpeg’) processImage(base64Image) } // code processing here return ( Capture Switch Camera ) } export default WebcamContainer When you click the Capture button the captureImage() function will capture the image on a specific video frame and send it for further processing. The full source code for the image capture user interface is in the WebcamContainer.jsx file. Color Palettes The color palette UI can be created using a series of dynamically colored svg rectangles. // eslint-disable-next-line react/prop-types const ColorRectangles = ({ colors }) => { return ( {colors.map((color, index) => ( ))} ) } export default ColorRectangles For example, if the colors array data is: [‘#4B8B3B’, ‘#C4B600’, ‘#7D7D7D’, ‘#E3D4A0’, ‘#2E2E2E’, ‘#F6F1D3’, ‘#A6A6A6’] Then the colors will be rendered on the web as the screenshot below: Server Routes The are four server routes to handle the client request. POST /process-image Process an image for color analysis. GET /colors The /colors route will retrieve all data from the database. GET /colors/:id Retrieve stored color data based on the ID. The data response for the picture field is a Buffer type so to process it in the browser, we need to change it into a readable format first. /** * Extracting the buffer data * Assume the result data name is jsonData */ const bufferData = jsonData[0][1].data; // Converting buffer data to Uint8Array object const uint8Array = new Uint8Array(bufferData); // Converting Uint8Array to UTF-8 string const utf8String = new TextDecoder(‘utf-8’).decode(uint8Array); console.log(utf8String); GET /delete/:id Delete specific data in the database by its ID. For example, to delete data with id 8900: http://localhost:3000/delete/8900 Tools like Postman can be used to test APIs. SQL Data Test To check the data in the database, we can use CLI commands. In this project, we use Ubuntu 20.04 LTS. Login to the GridDB user: sudo su gsadm and then type this command to enter the GridDB shell: gs_sh In this shell, we can list all containers and run any SQL queries. gs[public]> showcontainer gs[public]> select * from ColorPalettes; 1 results (38ms) gs[public]> delete from ColorPalettes where
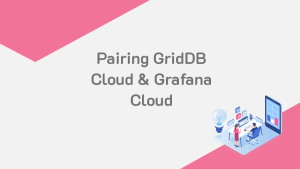
With the release of a completely free GridDB Cloud, we wanted to pair its free service with Grafana Cloud, another free Cloud-based service which can get you up and running in seconds. For this article, we will walk through the steps of how to display time-series data from your GridDB Cloud shared instance to Grafana Cloud. If you are unfamiliar with GridDB Cloud, you can read our quick start guide here: GridDB Cloud Quick Start Guide — that article will teach you how to sign up, how to begin using GridDB Cloud and more of the basics: who, what, when, where, why. If you are also unfamiliar with Grafana, you can read about its capabilities and strength from their docs: https://grafana.com/docs/grafana/latest/introduction/. If you are curious as to how Grafana can enhance your GridDB experience, I will point you to a previous article we have written here: (Creating Heatmaps of Geometry Data using Grafana & GridDB)[https://griddb.net/en/blog/creating-heatmaps-grafana/]. Essentially, Grafana’s advanced visualization tools allow for some creative ways of analyzing and peering into your data. This article’s goal is simple: showcase how to use the cloud offerings from both vendors to display some GridDB Data in Grafana Cloud. We will go through this process step-by-step and explain any idiosyncrasies along the way. Let’s get started! Implementation First, here’s a link to the Grafana dashboard that we will be using for this article: https://imru.grafana.net/public-dashboards/8a9f9f8ed9d34582aecca867a50c9613. Source code can be found here: $ git clone https://github.com/griddbnet/Blogs.git –branch 4_grafana_cloud Prereqs To follow along, you will need to have access to a free account of both GridDB Cloud and Grafana Cloud. Technical Overview To query our GridDB Cloud data from Grafana, we will be sending HTTP Requests directly from Grafana to our GridDB Cloud. And indeed, any sort of interactions we want to make with our free GridDB Cloud instance will be done via Web API interface; this topic is covered in the quick start linked above, as well as in this article: GridDB WebAPI. You can also of course check out the official docs: GridDB_Web_API_Reference. The specifics of how to form our query and how to create our allowlist to get around GridDB’s firewall will be the subject of our next few sections. Adding Grafana’s IP Addresses to GridDB’s Allowlist In order for our Grafana Cloud instance to send HTTP Requests which are accepted as “safe” by our GridDB Cloud, we need to be able to add all potential IP Addresses to our GridDB Cloud instance. Browsing through the Grafana documentation, we see that they have these lists readily available for these exact scenarios: https://grafana.com/docs/grafana-cloud/account-management/allow-list/. The list we need is called ‘Hosted Grafana’, and if you take a quick peek, you’ll see the list is easily over 100 lines, so then how do we efficiently add all of these to our GridDB Cloud management portal? Well, luckily we have already encountered this scenario in our previous article: Create a Serverless IoT Hub using GridDB Cloud and Microsoft Azure. To solve the issue, we wrote a simple bash script which will take the .txt file as the input and add each ip address to the allowlist of GridDB Cloud’s online portal. Source code and instructions found in that blog in the “#whitelist” section. Here’s the script: #!/bin/bash file=$1 #EXAMPLE #runCurl() { #curl ‘https://cloud57.griddb.com/mfcloud57/dbaas/web-api/contracts/m01wc1a/access-list’ -X POST -H ‘User-Agent: Mozilla/5.0 (Macintosh; Intel Mac OS X 10.15; rv:133.0) Gecko/20100101 Firefox/133.0’ -H ‘Accept: application/json, text/plain, */*’ -H ‘Accept-Language: en-US,en;q=0.5’ -H ‘Accept-Encoding: gzip, deflate, br, zstd’ -H ‘Content-Type: application/json;charset=utf-8’ -H ‘Access-Control-Allow-Origin: *’ -H ‘Authorization: Bearer eyJ0eXAiOiJBY2Nlc3MiLCJIUzI1NiJ9.eyJzdWIiOiJkMTg4NjlhZC1mYjUxLTQwMWMtOWQ0Yy03YzI3MGNkZTBmZDkiLCJleHAiOjE3MzEwMTEyMTMsInJvbGUiOiJBZG1pbiIsInN5c3RlbVR5cGUiOjF9.B1MsV9-Nu8m8mJbsp6dKABjJDBjQDdc9aRLffTlTcVM’ -H ‘Origin: https://cloud5197.griddb.com’ -H ‘Connection: keep-alive’ -H ‘Referer: https://cloud5197.griddb.com/mfcloud5197/portal/’ -H ‘Sec-Fetch-Dest: empty’ -H ‘Sec-Fetch-Mode: cors’ -H ‘Sec-Fetch-Site: same-origin’ -H ‘Priority: u=0’ -H ‘Pragma: no-cache’ -H ‘Cache-Control: no-cache’ –data-raw $1 #} runCurl() { $1 } while IFS= read -r line; do for ip in ${line//,/ }; do echo “Whitelisting IP Address: $ip” runCurl $ip done done < "$file" Querying GridDB Cloud from Grafana Cloud Out of the box, Grafana does have a method of sending HTTP Requests to your services, but from what I could tell, they're geared towards specific services (ie. Prometheus) and are limited to HTTP GET Requests. In our case, all of our requests to GridDB Cloud require POST requests, so we needed to find a solution for this: enter the Infinity plugin. Once installed, we will be able to add it as a datasource. Using Infinity as a Data Source From within your Grafana Cloud instance, select Connections --> Data Sources. If you installed the Infinity data source properly, it should show up in this section as an option — please select it. From here, we can add all of our pertinent GridDB Cloud information to forge our connection — we will need to add our basic authentication, and allow our portal’s hostname in the allowed hosts list. Once added, lastly click on the health check section and add your Web API + “/checkConnection” (ie. https://cloud5197.griddb.com:443/griddb/v2/gs_clustermfcloud5197/dbs/ZV8YUlQ8/checkConnection) as a simple sanity check AND health check. Hit Save & Test. We should be able to query our GridDB Cloud Database now! Ingesting Usable Time Series Data Before we query our data, let’s first ensure that we have working data in our GridDB Cloud. If you are following along and have just now made a new account, you can follow our quick start guide to ingest an IoT sample data that can be found on Kaggle. Here is a direct link to the section in the guide: https://griddb.net/en/blog/griddb-cloud-quick-start-guide/#ingest. Here, we are ingesting a csv file and calling the container device1. Forming Our HTTP Requests Now that we can communicate between services, let’s get the data we want. From the Grafana Cloud menu, select Dashboards and then select “new” in the top right corner and then finally Add visualization. From here, select Infinity and you will now have a blank graph and a place to put your query. And now for some options: Type: JSON Parser: Backend Source: URL Format: Data Frame Method: POST Note: Here is a screenshot of the entire query we will form in the ensuing lines of text (screenshot will be displayed again at the end once you can better understand what all of the words mean) The parser and format being what they are allows for us to properly name and label the data being received from GridDB Cloud because of the unusual way in which responds to the requestor with data. Instead of sending back the rows of data in JSON format (which, to be fair, if you’ve got a thousand rows, it’s a lot of unnecessary bloat), GridDB Cloud sends back the information as a JSON file, but the actual rows of data are in array form, with the schema being listed under another JSON key name (columns). As for the URL, you can take a look at the links above about how to form your Web API Request, but here are two we will be using: SQL: https://cloud5197.griddb.com/griddb/v2/gs_clustermfcloud5197/dbs/ZV8YUlQ8/sql/dml/query API: https://cloud5197.griddb.com:443/griddb/v2/gs_clustermfcloud5197/dbs/ZV8YUlQ8/containers/device1/rows In each case, we will have different selectors for our returned data, as well as different body payloads that we will be sending off as a request. First, let’s take a look at the SQL Query. Making a Simple SQL-Select Query First, set your URL to match the query above. The format is as follows: https://[cloud-portal-name].griddb.com/griddb/[clustername]/dbs/[database-name]/sql/dml/query. And then we form our SQL Query within the body of the request. To properly parse this, we set the format as data frame, and then under parsing options & Results fields, we type in “results” in the JSONata/rows selector box and then click add columns. To start, we simply want a couple of columns from our dataset, so we can se the columns like so: Selector: 0 #array index as: ts format-as: Time And then we select the column we want to track in our graph, let’s take a look at temperature Selector: 7 as: temperature format-as: Number Lastly, because it’s a POST request, we must send off something within the body of our request, but in this case, it’s going to be a SQL query. Right under the Method dropdown menu, there’s a button that says Headers, Body, Request Params. Click this. Fro that sub menu, set the Body Type to Raw and set the Body Content Type to JSON. And in the large text box you can add your actual body — or in our case, our SQL query: [ {“stmt” : “select * from device1 LIMIT 1000”} ] Note: I highly recommend using a limit on your query, otherwise Grafana may malfunction trying to show all of your data points. In the graph above, click “zoom in on data” if necessary and your data will be displayed! Cool! More SQL Queries (Group By Range, Aggregations) With the advent of GridDB 5.7, you can make some more complex SQL Queries as well and are not limited to SELECT statements. For example, we can use the SQL Group By Range which allows for us to perform a aggregation operations over a given time span. For example, this is what our query looks like: select ts,temp from device1 WHERE ts BETWEEN TIMESTAMP(‘2020-07-12T01:00:25.984Z’) AND TIMESTAMP(‘2020-07-12T01:22:29.050Z’) GROUP BY RANGE (ts) EVERY (1, SECOND) FILL (LINEAR). So we can simply plug this in to our Infinity plugin and see the results (Hint: you may need to change your column selectors and refresh the graph). You can also do other SQL aggregation queries, really there is no limit; you can read more about that in the GridDB SQL docs: https://griddb.org/docs-en/manuals/GridDB_SQL_Reference.html Using the Group By Range feature is excellent for creating dense graphs even if you don’t have a dense enough dataset! API Query We can also skip SQL and just the API to make queries using simple JSON options in our body request. The URL will be built out as follows: https://[cloud-portal-name].griddb.com/griddb/[clustername]/dbs/[database-name]/containers/[container-name]/rows So enter in your URL and, set the Body Content Type to JSON, and the Body content to some combination of the following: { “offset” : 0, “limit” : 100, “condition” : “temp >= 30”, “sort” : “temp desc” } As you can see, you set the condition and sort options for your dataset. The column options remain the same, except the results of the data is now called ‘rows’, so change that option in the Parsing Options & Result Fields section; you can keep the same column selectors as those stay the same. Conclusion Once you are done querying and adding in the data you like, you can of course save your dashboard for future use. And that’s all! If you have followed along, you have now been able to pair GridDB Cloud with Grafana Cloud to display your